Limit data bias in AI with healthcare-trained models
Report data and share feedback insights for a customer-centric strategy with a industry-specific, proprietary AI platform.
Get insights with Authenticx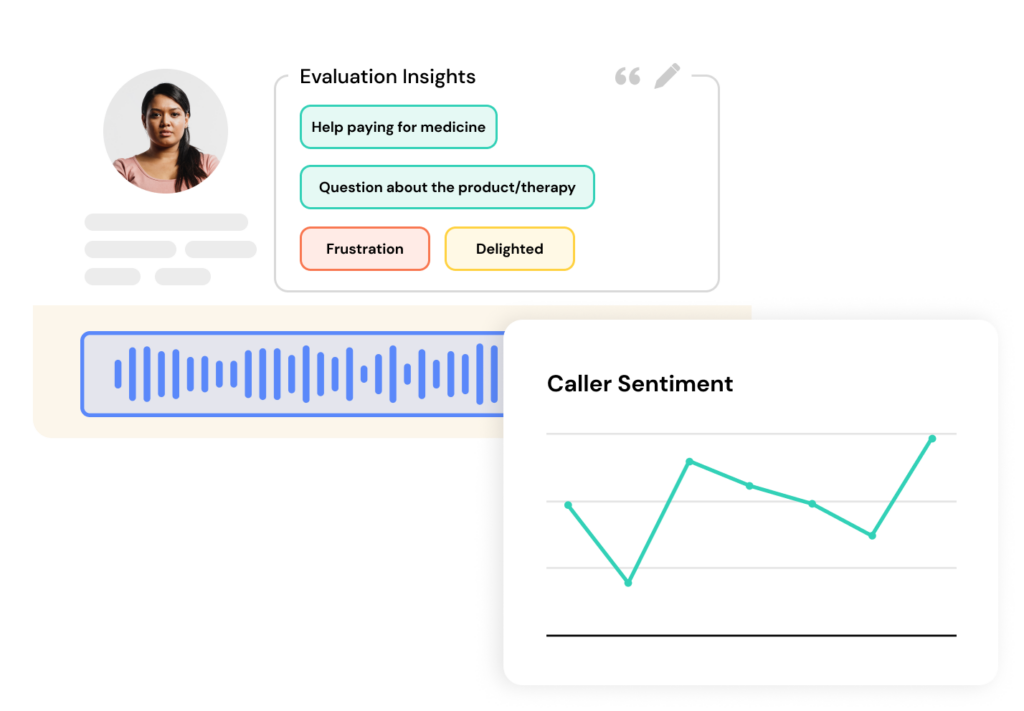
Break down the best practices for leveraging AI in your organization by following the impact of four customer stories. Download AI Best Practices →
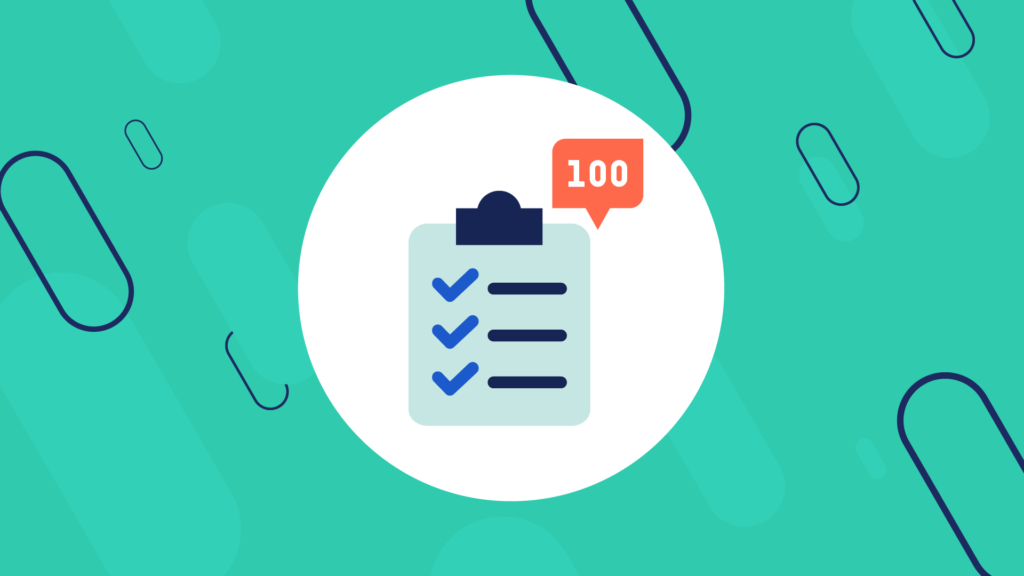
Increase Quality Score Across Call Center Vendors
With Authenticx insights, a life science enterprise eliminated self-reporting bias and received an reliable measurement of agent performance.
Read the Customer StoryData Bias in AI
AI has come a long way in recent years, transforming the way we interact with digital systems—and the world at large. Despite its gains, this technology is not without its downsides. This can show in the form of data bias in AI. Data used to train AI systems often contains biased or unrepresentative information. This can lead to the AI system itself reflecting or even amplifying these biases, resulting in unfair or incorrect outcomes.
Data bias in AI refers to the skewed or incomplete representation of information within AI training data. Such bias can arise from various sources, such as the collection process, preprocessing, or from inherent biases in human behavior captured in the data. It can lead to systematic errors in predictions or decision-making by the AI system.
For example, if a facial recognition system is trained predominantly on images of people from a specific ethnic background, it may perform poorly on recognizing faces from other ethnicities. This is an example of bias in AI, and it can result in misidentification or exclusion of certain groups.
This is especially problematic in sectors like healthcare, where data bias can have life-altering consequences. If a diagnostic model is trained on data predominantly from one demographic group, it may fail to recognize symptoms or make incorrect diagnoses for individuals outside of that group. While AI in healthcare can be used for good, it’s important that models are trained for the utmost accuracy.
Addressing bias in artificial intelligence in health care involves carefully curating and preprocessing the data, as well as being transparent about the algorithms, methodologies, and underlying assumptions. Some of the most common techniques used to solve these issues are oversampling underrepresented groups and employing human-in-the-loop strategies.
As tools and techniques evolve, bias is likely to become an issue of the past. In the meantime, however, it remains a forefront challenge—one businesses are increasingly trying to tackle in order to reduce discrimination.
Bias and Discrimination in AI
Bias and discrimination in AI can translate into real-world bias. For instance, if a job recruitment AI is trained on data that primarily includes male candidates, it may unfairly favor men in the hiring process, perpetuating existing inequalities. This shows how gender bias in data can lead to systemic discrimination against women.
Consider, too, an AI system that’s biased against applicants from lower-income neighborhoods. If the data used to train the AI incorporates past decisions where loans were denied to these individuals, the AI may continue this pattern. These AI discrimination examples demonstrate the seriousness of artificial intelligence bias.
So what is one potential cause of bias in an AI system? The number one cause of bias in AI systems is the data they’re trained on. If data is unrepresentative of the broader population or reflects existing human biases, these biases will be carried over into the AI system’s decision-making.
How can a computer program’s bias become dangerous? Because AI systems are increasingly utilized in critical decision-making processes like hiring, lending, policing, and healthcare, individuals may be unfairly denied the services they need to survive and prosper.
Types of Bias in AI
There are many different types of bias in AI. Understanding the various types of bias in machine learning and AI is crucial to improving data and, ultimately, eliminating discrimination from the equation. Here are some of the most common forms of bias:
- Algorithmic Bias: Algorithmic bias in AI occurs when the computational methods and algorithms used in AI inadvertently favor or discriminate against certain groups or characteristics.
- Gender Bias: Gender bias in AI results in the unfair favoring of one gender over the other, often reflecting societal stereotypes and biases in the data used to train AI systems.
- Sample Bias: One of the most widespread types of bias in data analysis, sample bias happens when the data collected is not representative of the population it’s meant to represent, leading to biased conclusions.
- Preprocessing Bias: Arises when the techniques used to clean and prepare data introduce errors or skew the information in a way that affects the AI’s decision-making.
- Measurement Bias: Measurement bias is a type of bias resulting from flawed data-collecting tools or methods. It can produce systematic errors in the data.
- Cultural Bias: Results when an AI system reflects the cultural norms and values of the group that designed or trained it, potentially leading to misunderstandings or exclusion of other cultural groups.
- Societal Bias: This is the reflection of broader societal biases, such as racism or sexism, within AI systems, often stemming from biased data or biased human decisions in the development process.
- Evaluation Bias: A type of discrimination in which the methods used to evaluate an AI system’s performance are biased, leading to incorrect assessments of how well the system is working.
- Labeling Bias: Occurs when the labels used in supervised learning are incorrect or biased, leading to incorrect predictions or classifications by the AI system.
By studying bias and fairness in machine learning, AI trainers and businesses alike can work to address the issues stemming from systematic bias and create a fairer digital world.
How It Works
Gain a deeper level understanding of contact center conversations with AI solutions.
Learn MoreAggregate
Pull customer interaction data across vendors, products, and services into a single source of truth.
Analyze
Collect quantitative and qualitative information to understand patterns and uncover opportunities.
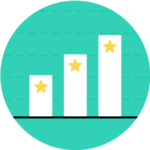
Activate
Confidently take action with insights that close the gap between your organization and your customers.
Examples of AI Bias in Healthcare
The presence of bias in AI is a critical issue that has manifested in various real-world scenarios, leading to skewed and sometimes harmful outcomes. Viewing examples of AI bias examples is key to understanding why these issues exist and determining how to go about addressing them.
Bias in machine learning examples includes sentiment analysis, which has shown biases against specific dialects or colloquial language. Similarly, certain facial recognition systems have demonstrated racial bias, performing less accurately on individuals from some racial groups.
Examples of AI bias in healthcare also abound. For instance, a heart disease prediction model trained mostly on male patients might overlook symptoms or risk factors more common in women. This can lead to poor outcomes—and even increased mortality—in patients, illustrating the danger of gender bias in machine learning.
These AI discrimination examples barely scratch the surface of bias in AI. As technology evolves over time, models will need to be trained on improved data that’s representative of the entire population. This can help prevent discrimination, especially in critical fields like healthcare.
Mitigating Bias in Artificial Intelligence
So what is bias mitigation, and how can it be applied to AI? Bias mitigation is the process of identifying, understanding, and reducing or eliminating biases in AI systems, ensuring that they function fairly and equitably. Here’s how to make AI less biased:
- Collect Diverse and Representative Data: Ensure that training data is inclusive. This can reduce, if not totally eliminate biases against particular groups.
- Promote Transparent Model Development: Being open about how a model works can aid in identifying and correcting biases.
- Incorporate Fairness Metrics: Utilizing metrics that explicitly measure fairness can help in maintaining balance in predictions or classifications.
- Account For Ethical Considerations in Development: Considering ethical principles during the development phase can lead to more responsible and fair AI systems.
- Engage Diverse Development Teams: A development team with varied backgrounds and perspectives can reduce the risk of unconscious biases influencing the AI’s design.
- Implement Regulatory Compliance: Complying with regulations that enforce fairness can act as a safeguard against biased practices in AI.
- Provide Education and Training for Practitioners: Training AI developers on biases and how to avoid them is vital to promoting more responsible AI development.
- Utilize Bias-Detection Tools: Using automated tools specifically designed to detect biases in AI models can assist in timely identification and correction.
Learning from mitigating bias examples is another great way to reduce bias. Look for flaws in other systems and see what developers have done to correct them.
Authenticx is also playing a role in mitigating bias in artificial intelligence by allowing users to holistically analyze customer voices. This customer experience software makes it possible for businesses to gain critical insights that are representative of all those they serve.
AI Bias Statistics
AI bias is an evolving and complex issue that has attracted significant attention in various fields. Recent AI bias statistics have revealed underlying biases in machine learning models that can lead to unequal and unfair outcomes. For example, a recent study found that over 30% of “facts” generated by AI are biased in some way.
AI bias and discrimination often stem from the training data that AI systems rely on, which may be skewed or unrepresentative of the broader population. Such biases can have real-world consequences, manifesting in discriminatory practices, such as favoring one demographic group over another in recruitment or loan approvals.
Perhaps most troubling is AI bias in healthcare. Medical algorithms trained on data that underrepresent certain racial or ethnic groups can lead to incorrect diagnoses or inappropriate treatment recommendations. Biases in healthcare data often result in unequal access to healthcare services, putting certain communities at a disadvantage.
One medical algorithm was found to be racially biased against black people. Only 18% of the patients it identified as needing additional care were black, with the additional 82% being white. Had the algorithm correctly identified the sickest patients, the numbers would have been 46% and 53% respectively.
The pursuit of unbiased AI is driving a significant amount of AI bias research. This research will hopefully lead to better outcomes for all, whether in healthcare, lending, or anything in between. The relationship between AI and gender equality—as well as other forms of equality—could not be more apparent, which is why it has become more critical than ever to develop better models.