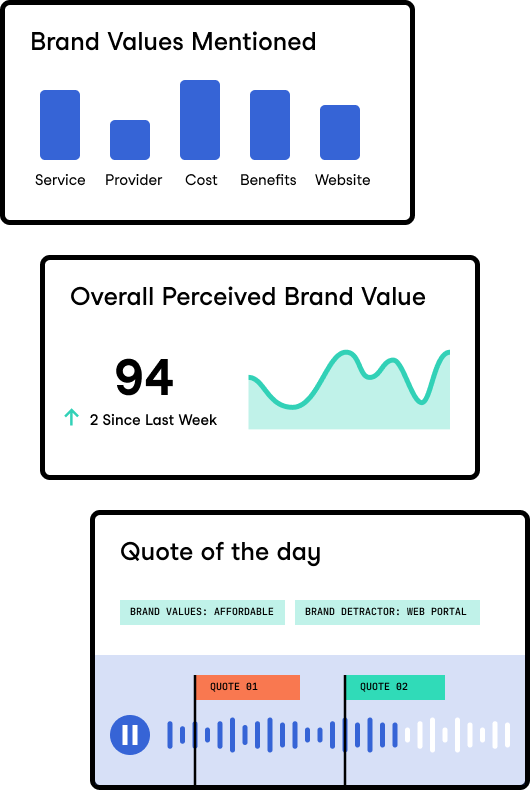
Leverage NLP in Pharma to unlock driven insights built from healthcare
Authenticx generates NLU algorithms specifically for healthcare pharma to share immersive and intelligent insights.
Unlock Conversations with AIMyths vs. Facts: How Artificial Intelligence Is Changing the Way Healthcare Listens
Software that connects qualitative human emotion to quantitative metrics.
Collecting data is only part of the equation. Understanding begins by listening and engaging with the story your customers are sharing.
See Authenticx in Action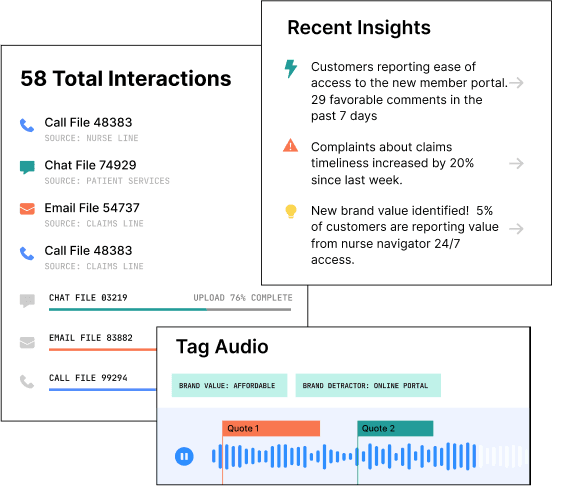
NLP in Pharma
Along with new technologies, the pharmaceutical industry is transforming at breakneck speed. By utilizing smart technologies such as AI, NLP in Pharma and deep learning, pharmaceutical companies can leverage big data and other data sources.
Natural language processing, also known as NLP, can be used to optimize pharmaceutical data by utilizing machine learning for data analytics, data comparison, and answering common questions.
What is NLP? A subset of artificial intelligence, or AI, NLP is a technique that can be used for decoding, analyzing, and manipulating human speech and text. NLP combines linguistics and computer science to make machines understand natural human language.
Regarding the application of NLP in pharmaceutical industry, NLP can be used to evaluate the market potential of a newly developed medicine or to more precisely target patients for existing medications. In the pharmaceutical and biotech industries, NLP can be useful for drug development, clinical trials, and for improving commercial engagement and sales productivity.
Email spam detection is a natural language processing example. NLP can detect specific keywords or topics in incoming messages, such as those associated with spam emails, phishing, and social engineering entrapment. Another NLP example includes text prediction, which includes autocorrect and auto complete functions. NLP can also help marketers and market researchers detect core issues, perform sentiment analysis, and identify keywords and key phrases in unstructured datasets derived from customer interactions.
As for natural language processing example projects in the healthcare industry, NLP can be used to extract information on clinical notes. NLP can process clinical notes to convert unstructured text into structured data elements by pulling out medical conditions, drug treatment and diagnostic procedures. NLP in Pharma can also automatically identify and extract relevant information from radiology reports.
Authenticx is a software company that uses artificial intelligence to help healthcare organizations analyze and understand customer interaction data. To improve customer experience, Authenticx can bring the voice of customers to decision-makers. Using AI and natural language processing, Authenticx can assist healthcare organizations in extracting insights from recorded customer interactions such as calls, emails, or chats. This can enable healthcare providers to develop a more personal relationship with their patients by listening to what matters most to them.
Pharmacovigilance
According to the World Health Organization, pharmacovigilance is the science and activities relating to the detection, assessment, understanding, and prevention of adverse effects or any other medicine or vaccine-related problem. That is where NLP in pharmacovigilance comes into play.
For other usage examples of NLP in Pharma, drug safety monitoring is one of them. Using natural language processing to help monitor reactions to drug administration is a cost-effective way to detect and respond to adverse reactions more quickly. This can enable healthcare organizations to save money and can make the healthcare system safer for both patients and practitioners.
It can be challenging for pharma brand leaders to quickly interpret their customers’ experiences and make the necessary adjustments to address customer concerns about drug and service quality. Market research data can only provide so much direct insight. The reporting of adverse drug events remains a regulatory concern as pharma companies manage an expanding omnichannel strategy, with a constant stream of conversational data to handle.
A software tool such as Authenticx can help healthcare organizations in need of resources to listen to and analyze customer interactions at scale on a regular basis. Authenticx uses artificial intelligence and natural language processing to skim through large volumes of customer interactions and highlight the most important parts. Authenticx can help pharma organizations aggregate their customers’ voices in real time so that they can analyze them to gather insights that they can use to improve their products or services.
Another natural language processing example would be in the pharmaceutical industry, NLP can assist healthcare executives in identifying and segregating valuable insights from their existing data. NLP and other machine learning tools can make it easier to categorize and organize large amounts of data that were previously difficult to locate, evaluate, and share with the relevant people.
NLP can also be used to assist researchers in the fight against the COVID-19 pandemic. NLP in Pharma can evaluate incoming email and live chat data from patient help lines to identify those who may have COVID-19 symptoms. This can allow doctors to prioritize those who may require assistance and get patients to the hospital more quickly.
Healthcare Analytics
Among the benefits of NLP in healthcare is that NLP can be used to improve patients’ health literacy. Health literacy refers to patients’ ability to obtain, understand and use health information to make informed healthcare decisions. While natural language processing cannot replace medical professionals, NLP can be used to allow patients to interact with healthcare chatbots. The use of healthcare chatbots can improve communication between patients and health professionals. Healthcare chatbots can also provide an immediate response to patients’ healthcare-related queries.
There are many other possible applications for NLP. Healthcare companies use NLP to gain an understanding of the massive amounts of unstructured data contained in electronic health records and provide patients with more quality healthcare.
In healthcare, NLP datasets can be enormous. As the healthcare industry becomes more digitized, clinical text data generated from multiple channels such as online portals, PDF reports, emails, text messages, and chatbots is unstructured and difficult to analyze. NLP can enable healthcare organizations to obtain health insights from data points. Unstructured clinical text can be extracted, codified, and stored in a structured format for analysis using NLP methods.
Despite the benefits of NLP in healthcare, among the challenges of NLP in healthcare is the complexity of terms used in the field. For example, different definitions can exist for the same terminology. The healthcare industry also uses abbreviations that may be ambiguous for NLP.
Life Sciences Speech Analytics
There are various applications of natural language processing in healthcare and biomedicine, especially for NLP in life sciences. Healthcare organizations use NLP to organize medical records and patient data so they can be analyzed more easily. The use of NLP in drug discovery can help organize massive amounts of data generated during the process. In the field of medicine, NLP can be used to analyze customer feedback on medications so that pharmaceutical companies can address drug-related issues more effectively.
Life sciences companies can use NLP to extract value from unstructured data and generate knowledge from a variety of sources. Documents collected in various locations are frequently stored in silos, resulting in bottlenecks. Issues regarding unstructured data can be addressed by creating a central database of knowledge and using technology to bring all of that data into a central location for analysis.
Authenticx is on a mission to help people better understand one another. Authenticx collects data from recorded customer interactions such as phone calls, emails, and chats and assists healthcare companies in extracting useful information from customer conversations.
Using AI and NLP, Authenticx gathers information for all parts of the healthcare enterprise, allowing healthcare leaders to make better business decisions. Authenticx provides a platform that allows healthcare executives to interact with their customers’ voices.
How It Works
Gain a deeper level understanding of contact center conversations with AI solutions.
See a PreviewAggregate
Pull customer interaction data across vendors, products, and services into a single source of truth.
Analyze
Collect quantitative and qualitative information to understand patterns and uncover opportunities.
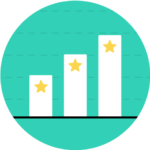
Activate
Confidently take action with insights that close the gap between your organization and your customers.
NLP in Pharma and Biotech
There is much to consider when it comes to NLP in biotech. Installing the NLTK, or Natural Language Toolkit, on a system is required for using NLP. NLTK refers to a collection of symbolic and statistical natural language processing programs written in Python programming language. In the case of medical NLP, Python programs can be used to make sense of natural language processing examples. Python is a computer programming language that is used to build websites and software, automate tasks, and conduct data analysis.
NLTK is a leading platform for building Python programs to work with human language data. NLTK is widely used for natural language processing and has a large corpora and lexical resources. NLTK has a suit of text libraries for classification, or categorizing text into a group of words; tokenization, or breaking up raw text into words; stemming, or reducing a word to its base form or root format; tagging, or categorizing words in a text in correspondence with a particular part of speech; and many others.
Clinical Trials
Regarding the connection between artificial intelligence and clinical trials, as well as NLP in clinical trials, AI technology, combined with big data, can be used to streamline the processes of clinical trials. By using a clinical trial matching AI platform, healthcare organizations may be able to increase clinical trial enrollment and reduce the screening period in clinical trial matches.
In healthcare, there are many benefits of using natural language processing. Healthcare companies can use NLP to develop treatment plans. NLP can also enable healthcare practitioners to automatically review massive amounts of unstructured clinical and patient data to identify suitable candidates for clinical trials.
NLP Solution
Text analytics for health industry and text analytics use cases enable the retrieval and classification of relevant medical information from unstructured texts. Unstructured texts include doctor’s notes, patient treatment records, clinical documentation, and electronic health records. Machine learning techniques such as NLP can be used to automate medical text analysis of patient records. NLP can be used to speed up the analysis of patient records files, deduce information from what is written on patient records, categorize files, and even add additional information on the specified medical conditions.
To leverage the power of natural language to provide quality healthcare, organizations may benefit by working with the best NLP solution providers to provide a viable NLP in Pharma solution.
Providing NLP solutions for healthcare, Authenticx platform unifies customer interactions from call centers, texts, chats, emails, and other channels into a single source of truth.
Organizations in the healthcare industry, such as pharmaceutical and health insurance companies, can organize and structure conversational data using a customized dashboard. Healthcare executives can use Authenticx to gain insights from patient data and make better business decisions.