AI bias in healthcare can impact your CX.
Healthcare conversations are different. The use of technology helps provide enormous insights and opportunities for organizations to unlock the how, when, and why.
See How Authenticx Helps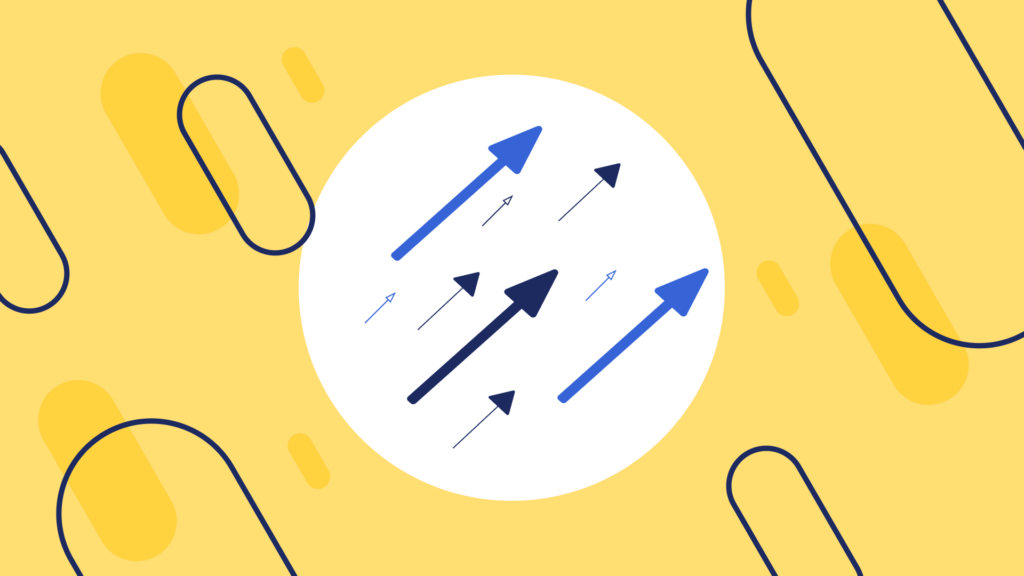
Authenticx analyzes customer conversations to surface recurring trends in unsolicited feedback.
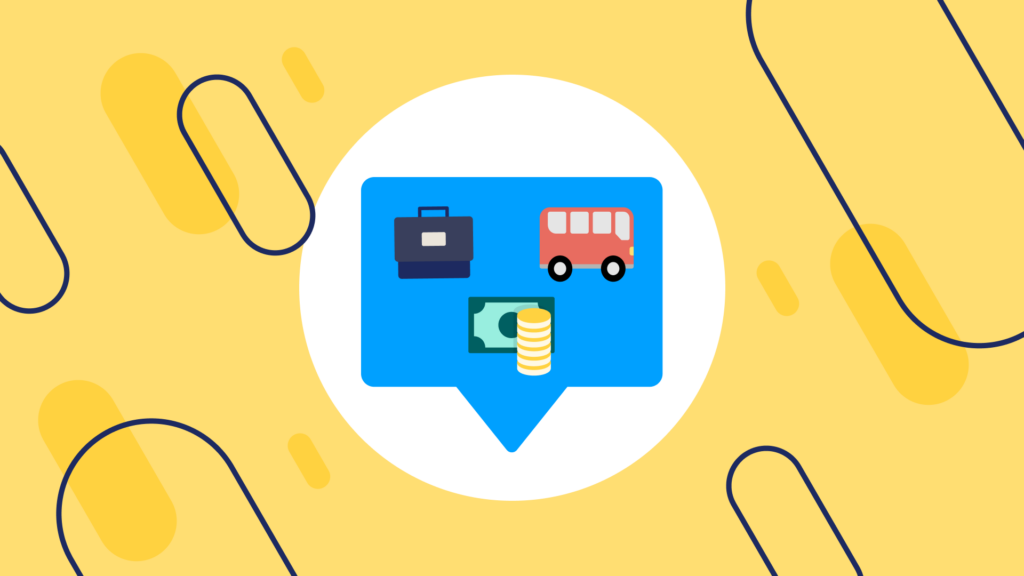
Identify disruptions and create impact with data insights.
Understand the patient experience by understanding the complexities of healthcare (including social determinants of health).
View Case StudyAI Bias In Healthcare
Artificial intelligence (AI) has been a transformative force across various industries, and healthcare is no exception. AI in healthcare projects has shown great potential in improving patient care, diagnostics, and treatment plans. AI also comes with its fair share of challenges, however, one of which is AI bias in healthcare.
AI bias refers to the systematic discrimination that’s often embedded within AI systems. This can result from flawed or biased data inputs, algorithms, or human decision-making. Algorithmic bias in healthcare can lead to disparities in treatment and health outcomes for certain populations, especially those already experiencing health disparities due to socio-economic, gender, or racial factors.
A pertinent question to ask is, “Are there areas of your life that can potentially be affected by algorithmic biases?” In the context of healthcare, the answer for most people is yes, to some extent. For example, AI bias in healthcare may manifest in diagnostic tools that disproportionately misdiagnose certain diseases in specific populations, leading to inadequate or inappropriate treatment.
AI in healthcare statistics reveals that a significant number of healthcare providers and organizations are adopting AI-powered tools and solutions. While AI has the power to improve healthcare outcomes, the presence of bias in these systems can undermine the benefits and lead to unintended consequences. As such, addressing bias in artificial intelligence in health care is of the utmost importance.
Considering the impact that bias can have on patient health outcomes, addressing bias in artificial intelligence in health care should be a top priority for all healthcare entities that utilize AI. To address these issues, stakeholders must engage in a multi-faceted approach. This includes:
- Increasing diversity in data sets: Ensuring that AI models are trained on diverse data can help reduce the likelihood of bias.
- Improving transparency and explainability: Healthcare providers must demand that AI system developers provide clear explanations of how their algorithms work, the data used to train them, and the potential biases embedded within them. This can help identify and mitigate biases before they impact patient care.
- Implementing bias-detection mechanisms: Regularly monitoring and auditing AI systems for bias involves developing robust and transparent methods to assess and quantify biases in AI algorithms.
- Encouraging interdisciplinary collaboration: Tackling AI bias in healthcare requires the collaboration of experts from various fields, including computer science, medicine, ethics, and social sciences. This interdisciplinary approach can help create more equitable AI systems that serve diverse patient populations.
What is AI Bias?
So what is AI bias? As discussed previously, AI bias refers to the presence of systematic errors in the output generated by AI systems resulting from inaccuracies or prejudices in the underlying data or algorithms.
Bias and discrimination in AI unfairly target and/or exclude specific groups based on factors such as race, gender, and class. This can be demonstrated through an AI system for hiring that exhibits bias against women because the training data primarily consists of successful male applicants.
The two main types of AI bias are data bias and algorithmic bias. Data bias occurs when the training data used to develop AI systems contains skewed or unrepresentative information. This can lead to biased predictions because the model will learn from and perpetuate the patterns present in the data.
Algorithmic bias, on the other hand, stems from biases inherent in the design or application of the AI algorithms themselves. It can occur due to oversimplified assumptions or the use of inappropriate performance metrics.
One prominent example of AI bias is predictive policing, a law enforcement practice that leverages AI algorithms to forecast crime hotspots and potential offenders. Predictive policing bias arises when biased data, such as historical arrest records, are used to train the AI models. This can result in an overemphasis on certain neighborhoods or demographic groups.
To raise awareness and promote understanding of AI bias, researchers have published numerous articles discussing the causes, consequences, and potential solutions for algorithmic bias. These algorithmic bias articles highlight specific instances of AI bias, such as racial bias in facial recognition systems or gender bias in natural language processing models.
By providing accessible information on AI bias, articles such as these aim to foster a broader public understanding of the issue, prompting the development of more equitable and inclusive AI systems.
AI Bias Examples
Viewing AI bias in healthcare examples can be a good way to learn more about what this issue entails. For instance, when it comes to digital discrimination in healthcare, diagnostic tools that rely on biased training data may produce less accurate predictions for certain racial or ethnic groups. AI bias examples like these demonstrate the need for better system training.
A great example of AI bias in healthcare is the use of predictive algorithms for patient risk assessment. It has been found that certain healthcare algorithms exhibit racial bias, systematically underestimating the healthcare needs of Black patients compared to white patients. This sort of bias limits access to healthcare resources and programs designed to improve patient outcomes.
Other algorithmic biases in healthcare examples include issues related to diagnosis. AI diagnostic tools often perform poorly on patients with darker skin tones due to a lack of diverse skin types in the training data. This can result in delayed or inaccurate diagnoses for patients from marginalized communities, increasing the risk of negative health outcomes.
But healthcare isn’t the only industry experiencing digital bias. AI bias in education is another challenge that must be addressed in order to ensure more positive outcomes for all. This is highlighted by AI-powered grading systems for evaluating student essays or other written assignments. These systems often rely on natural language processing (NLP) algorithms, which are trained on large datasets of text to learn patterns and assess the quality of writing.
AI bias can emerge in such grading systems if the training data predominantly consists of texts written by individuals from certain demographic or linguistic backgrounds. As a result, the AI model may develop a preference for specific writing styles, vocabulary, or sentence structures that align with those present in the training data.
How It Works
Gain a deeper level understanding of contact center conversations with AI solutions.
Learn MoreAggregate
Pull customer interaction data across vendors, products, and services into a single source of truth.
Analyze
Collect quantitative and qualitative information to understand patterns and uncover opportunities.
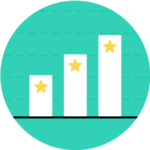
Activate
Confidently take action with insights that close the gap between your organization and your customers.
AI in Healthcare
AI in healthcare has the potential to revolutionize the way we approach medical care. Technological advancements offer numerous benefits, such as reduced costs, improved accuracy, and enhanced patient outcomes. However, implementing AI in healthcare comes with its own set of challenges and ethical considerations, including algorithmic bias and the need for transparency.
One major challenge in implementing AI in healthcare is ensuring the accuracy and reliability of algorithms. One of the most well-known algorithmic bias real-life examples is the use of AI to manage chronic kidney disease. Very often, these algorithms under-predict the need for additional care among certain demographics, which can lead to delayed care and the patients getting sicker.
Another challenge is ensuring the privacy and security of patient data. Because AI systems rely heavily on large datasets, the potential for data breaches and unauthorized access to sensitive information is a significant concern. Healthcare organizations must implement robust security measures and comply with data protection regulations to mitigate this risk.
Ethical considerations are paramount when implementing AI in healthcare, as the potential consequences of misuse can be severe. There is a risk that AI could be used to prioritize certain patient groups over others, leading to unfair treatment or resource allocation. Reliance on AI may also devalue the importance of human medical professionals, who possess empathy and the ability to make complex decisions that are unachievable using AI alone.
It’s important for researchers to study examples of AI bias in healthcare to better understand healthcare algorithms bias and determine the best course of action for addressing these problems.
Future of AI in Healthcare
The future of AI in healthcare promises to bring transformative change to improve the efficiency, accessibility, and quality of medical care. Going forward, we can expect advancements in precision medicine, telemedicine, and remote patient monitoring. AI-driven tools will enable personalized treatment plans tailored to each patient’s unique genetic and lifestyle factors.
Even today, though, bias remains a major concern, and the healthcare space especially must find ways to address problems with these technologies. Artificial intelligence, bias, and clinical safety are interconnected, and understanding this relationship is key to the successful implementation of AI in healthcare.
The recently-published article “Addressing Bias in Big Data and AI for Health Care: A Call for Open Science” highlights the need for increased transparency and collaboration in the development and deployment of AI tools in healthcare. “Artificial Intelligence and Algorithmic Bias: Implications For Health Systems” is another valuable article that explores the relationship between AI and ongoing systemic discrimination in healthcare.
Resources like these play a vital role in the development of new AI technologies. They help to foster a greater understanding of AI bias and how it can be addressed most effectively. Healthcare organizations should remain up to date with current research to ensure they’re following best practices for eliminating bias in AI systems. By doing so, they can promote a better future for all patients—one that treats patients as individuals and works closely with them to provide treatments that suit their specific needs.
Authenticx understands the importance of the human touch in healthcare. The platform helps to remove bias from processes by connecting qualitative human emotion to quantitative metrics. This sentiment analysis software yields more productive insights that can be used to address some of the most critical concerns in AI today.