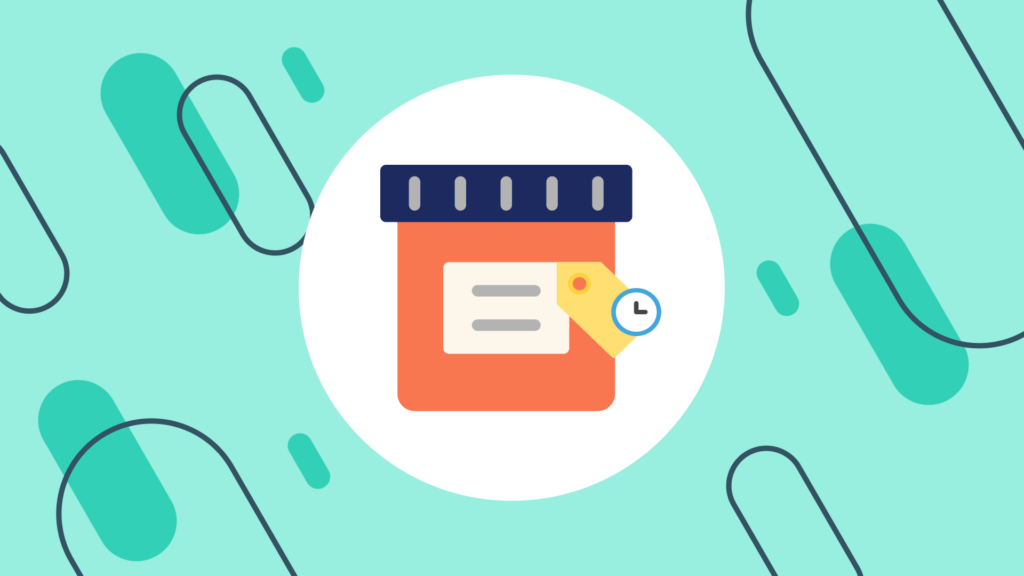
Leverage data analytics in the pharmaceutical industry with AI
Capture what call center specialists say to callers and understand what the patients were asking regarding updated policies.
See Authenticx in ActionAuthenticx analyzes customer conversations to surface recurring trends.
5 Ways Call Center Scripts are Important for Healthcare Conversations
Healthcare call center scripts are tremendously helpful for alignment on training, but it’s hard to assess actionable feedback on performance without the ability to listen at scale.
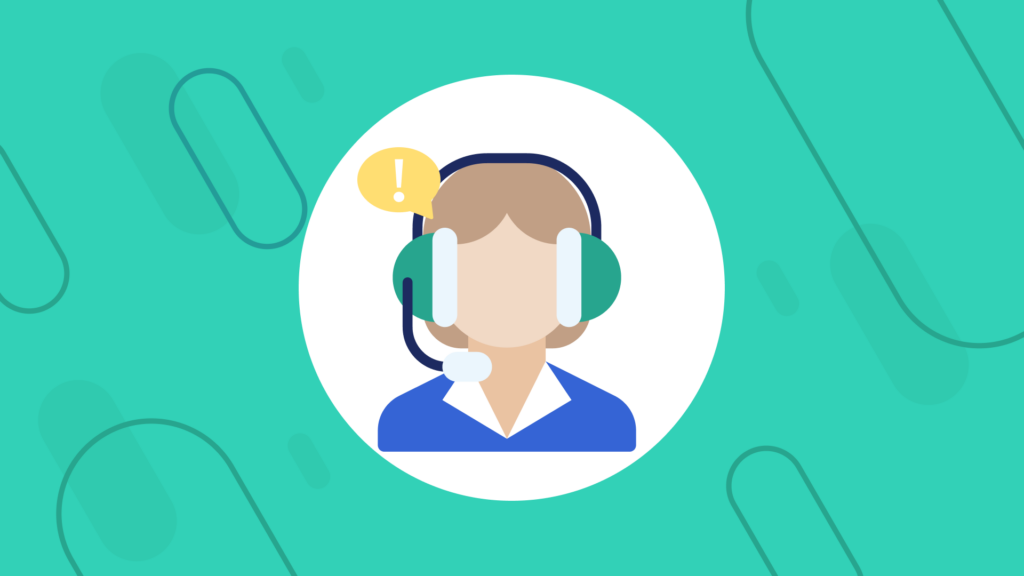
Data Analytics in Pharmaceutical Industry
As more and more companies find ways to improve operations to keep up with customer demand and stay ahead of the competition, data analytics is increasingly becoming a part of business operations in a variety of industries – and the pharmaceutical industry is no exception. Data analytics in pharmaceutical industry refers to the process of collecting data from various sources and analyzing or structuring that data to provide greater insights regarding pharmaceutical products, clinical trials, drug safety, as well as patient outcomes.
Big data analytics in pharmaceutical industry practices is revolutionizing the way new drugs are discovered, developed, tested, and even how they are brought to the marketplace. Business analytics in pharmaceutical industry applications can help by enabling the collection, analysis, and integration of large volumes of data from various sources like clinical trials, electronic health records (EHRs), and genomic data.
By leveraging modern advanced analytics technologies like machine learning and artificial intelligence, pharmaceutical organizations can more readily identify new drug targets, design more effective clinical trials, and even accelerate development process timelines. Big data analytics can also improve drug safety initiatives by identifying potential adverse reactions and medication interactions, which, in turn, reduces the risk of exposing patients to unnecessary issues.
Healthcare data analyst requirements typically include general knowledge of healthcare industry best practices as well as a combination of various technical and analytical skills. As a data analyst in pharmaceutical industry applications, your primary duties would likely involve collecting, analyzing, and attempting to interpret complex healthcare data in order to ultimately improve patient outcomes.
Healthcare data analysts should also have a comprehensive understanding of healthcare industry operations, and relevant industry and regional regulations, including knowledge of healthcare data standards, privacy regulations, and reimbursement technologies. Strong communication skills and collaboration experience are invaluable because healthcare data analysts must be able to communicate effectively with multiple teams about complex data insights.
As the technologies involved continue to improve, data analytics will remain an essential tool for professionals within the pharmaceutical industry. By collecting and analyzing data from various reputable sources, pharmaceutical companies can enhance the safety and effectiveness of their products while generally improving patient outcomes. Data analytics are also useful in the realm of pharmaceuticals because businesses can leverage data insights to support other aspects of business operations related to supply chain management, sales, and marketing. For the foreseeable future, data analytics will likely continue to play a significant role in helping pharmaceutical companies innovate and deliver safer, more effective treatment methods to patients.
Pharma Analytics
Data analytics in pharmaceutical settings is often shortened to ‘pharma analytics’, but the term generally refers to the same process of using data analysis techniques to analyze gross data sets within the pharmaceutical and healthcare industries. The underlying goal of pharmaceutical analytics seeks to extract meaningful insights from complex and diverse sets of data.
Another similar term, pharmacy informatics, refers to the application of information and data analytics to the realm of pharmaceuticals. It involves the use of computerized physician order entry (CPOE) systems, EHRs, medication management systems, and other healthcare tech in order to enhance pharmacy operations.
Pharma analytics necessitates the use of various analytical tools and methodologies. Tools like machine learning, natural language processing, predictive modeling, and data visualization are all great examples of techniques that empower pharmaceutical companies to identify patterns and correlations within data to improve various aspects of business operations.
Pharmaceutical analytics has several useful applications within the pharmaceutical industry, from drug discovery and development to personalized medicine, pharmacovigilance, market research, and others. By harnessing the power of data analytics, pharmaceutical organizations can expedite the drug manufacturing process, improve the safety and efficacy of various medications, and streamline workflows.
For people interested in developing their healthcare data analyst skills, there are a number of healthcare data analytics books that can provide a greater understanding of the concepts, tools, and techniques required to excel in the exciting field of healthcare data analytics. As more companies seek data to help improve decision-making and other aspects of business, data analysts will continue to play a vital role in helping organizations understand how to interpret their data.
Even if your business can collect more data than any other in the world, that data is largely useless without effective analysis personnel and data analytics specialists in place to provide invaluable insights.
Machine Learning in Pharmaceutical Industry
The future of data science in pharmaceutical industry applications looks, by all accounts, extremely promising. With the potential to transform the industry from the ground up, it’s unlikely that data science will fall out of vogue any time soon. Pharmaceutical companies that are able to take advantage of the many recent advancements in the realm of data science and machine learning technologies will be in a much better position than the companies that do not. Industry experts also predict that data science techniques will also contribute to improving healthcare operations and supply chain management, which is excellent news for patients and healthcare providers alike.
Machine learning in pharmaceutical industry settings can help improve drug discovery and development processes, optimize clinical trials, and even personalize patient treatments. Because machine learning technology utilizes advanced algorithms and statistical models to enable computer programs to “learn” from data and make predictions, this is incredibly useful within the pharmaceutical industry.
One of the primary benefits of utilizing machine learning technology within the pharmaceutical industry is the advantages in research and development. Machine learning algorithms can effectively identify potential drug targets, predict the efficacy and safety of certain drug candidates, and even optimize a medication’s design.
In addition to bolstering the pace and effectiveness of the drug discovery process, data analytics in pharma R&D can also help companies reduce costs and improve the success rates of clinical trials. However, R&D data analytics is oftentimes a complicated and expensive process that requires multiple steps. In the drug discovery stage, for example, data analytics helps companies expedite the process by analyzing large data sets. And in the clinical trial stages, data analytics helps by rapidly identifying patient subgroups that are more likely to respond to a particular treatment.
Overall, pharmaceutical data analytics is a great tool for improving patient safety and numerous other aspects of healthcare applications.
How It Works
Gain a deeper level understanding of contact center conversations with AI solutions.
Learn MoreAggregate
Pull customer interaction data across vendors, products, and services into a single source of truth.
Analyze
Collect quantitative and qualitative information to understand patterns and uncover opportunities.
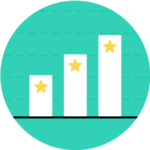
Activate
Confidently take action with insights that close the gap between your organization and your customers.
Pharmaceutical Data
Big data in pharmaceutical industry applications likely isn’t going anywhere anytime soon. In fact, there are practically innumerable types of data in pharmaceutical industry settings. Some of the most common types of pharmaceutical industry data include clinical trial data, electronic health records (EHRs), sales data, and genomic data.
Clinical trial data generally refers to information on the safety and efficacy of drugs currently in development, while EHRs more commonly provide information about patients – diagnoses, treatments, and patient outcomes. Sales data provides information on drug sales and current market trends, and genomic data refers to the information associated with a patient’s unique genetic makeup.
There are a variety of methods for collecting pharmaceutical data and constructing pharmaceutical data sets. Pharmaceutical companies can collect clinical trial data by receiving patient-reported outcomes, aggregating EHRs, and leveraging medical imaging modalities. Once the data has been gathered, machine learning algorithms are deployed to identify patterns and glean insights to better inform drug development procedures.
Many pharmaceutical companies already have large stores of data, it’s just that the data might be siloed or otherwise inaccessible. In these cases, it helps to implement effective data integration and management strategies to make the data more accessible.
Data Science in Drug Development
Pharmaceutical analytics companies have leveraged data science in drug development to improve both the efficacy and effectiveness of the drug development process. By relying on many of the advanced analytics techniques we have discussed in this article, pharmaceutical analytics companies can analyze large and complex sets of data to better understand disease mechanisms, identify potential drug targets, and predict with greater accuracy the safety and efficacy of drug candidates.
In more ways than one, data science projects in pharmaceutical industry settings are revolutionizing the entire field of healthcare. These projects often involve the collection and analysis of unfathomably large and complicated data sets from innumerable sources.
Together, a clinical data analyst and a pharmaceutical scientist can combine their areas of expertise to enhance the quality of clinical research in the pharmaceutical industry. With data science, know-how, and a deep understanding of the regulatory and compliance requirements governing clinical research, a data analyst can offer invaluable insight throughout the process. Similarly, pharmaceutical scientists bring their knowledge of drug development and clinical trial design to the table to identify potential drug targets.
Data science is changing the way the pharmaceutical industry approaches drug discovery, pre-clinical development, and clinical trial processes. By making the most of machine learning algorithms and other predictive modeling systems, healthcare scientists are now able to analyze gross volumes of data to identify potential drug candidates with unprecedented accuracy and efficiency.
Integrating data science into the drug development process means pharmaceutical companies can streamline operations while reducing overhead costs, ultimately benefiting patients and other stakeholders by accelerating valuable deliverables like effective new drugs and treatment methods.