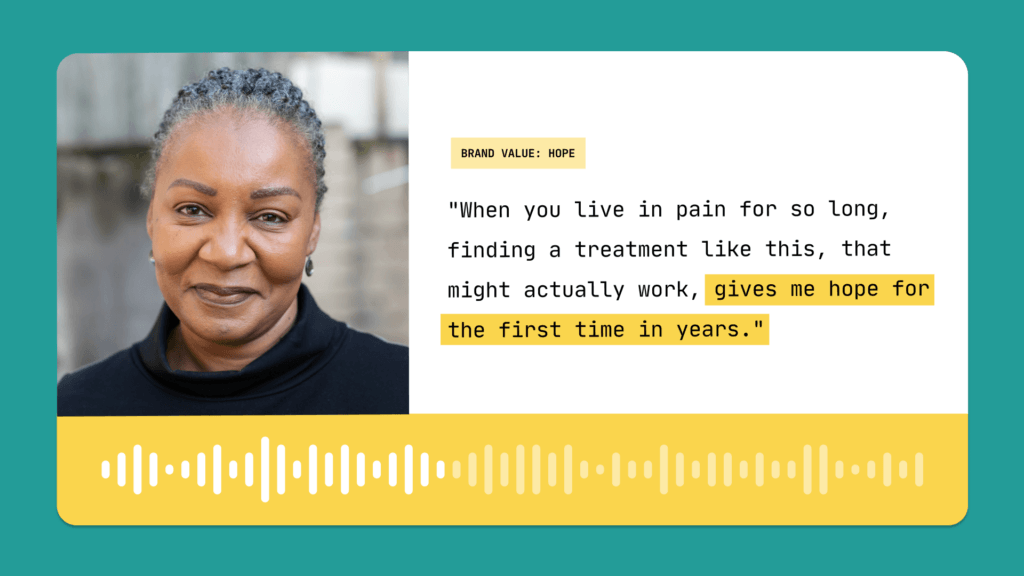
Insights that speak to the head and the heart.
Authenticx generates NLU algorithms specifically for healthcare to share immersive and intelligent insights.
Learn MoreAuthenticx analyzes customer conversations to surface recurring trends.
Software that connects qualitative human emotion to quantitative metrics.
Understanding begins by listening and engaging with the story your customers are sharing through insights discovered in data-backed storytelling.
See Authenticx in Action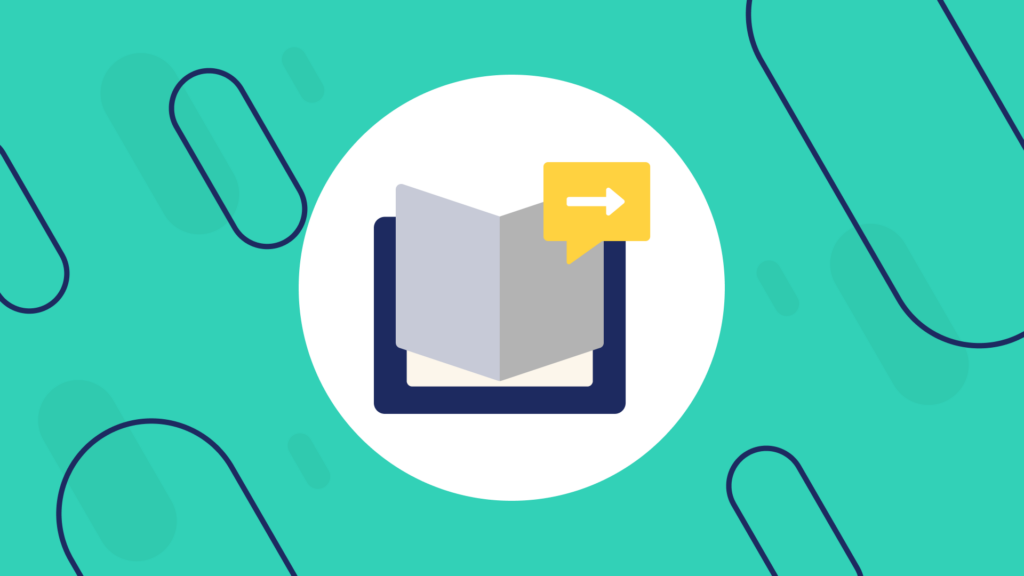
Text Sentiment Analysis Software
Text sentiment analysis is a type of natural language processing (NLP) software that uses machine learning algorithms to identify the emotional tone of written content, such as social media posts, customer feedback, or survey responses. The software analyzes the text to determine whether the sentiment expressed is positive, negative, or neutral. It can then provide a quantitative measure of the strength of the sentiment.
In the context of AI, text sentiment analysis software can be used to analyze large volumes of survey responses quickly and accurately. By identifying and categorizing the emotional tone of responses, the software can provide valuable insights into customer sentiment. These insights can help organizations improve their products, services, and customer experiences by identifying areas of strengths and weaknesses.
Prior to the introduction of sentiment analyzer software, analyzing survey responses was a time-consuming and labor-intensive process. It often required teams of analysts to manually read and categorize responses. With a text sentiment analysis tool, you can now analyze large volumes of survey responses quickly and accurately, reducing the time and resources required to derive meaningful insights from data.
In addition, this type of software can help organizations identify emerging trends and issues, such as changes in customer preferences or concerns about specific products or services. By spotting these trends early on, organizations can proactively address issues and improve their offerings to better meet the needs of their customers. This is key to promoting business growth and boosting revenue.
Sentiment analysis software offers many benefits, including:
- Improved customer insights:
- Sentiment analysis software can help businesses understand their customers better by analyzing their opinions, attitudes, and emotions. This can provide valuable insights into customer needs and preferences, which can be used to improve products, services, and customer experiences.
- Brand reputation management:
- Unlike sentiment analysis from scratch, automated sentiment analysis tools allow businesses to easily monitor their online reputation by tracking mentions of their brand on social media, review sites, and other online platforms.
- Marketing campaign optimization:
- Sentiment analysis can help businesses analyze the effectiveness of their marketing campaigns by tracking customer reactions and sentiment. By doing so, companies can optimize their campaigns to better reach their target audience and improve conversion rates.
Authenticx’s solution is built to help healthcare organizations identify issues that are impacting the customer journey. It does so by listening to and analyzing customer voices. With Authenticx, companies can obtain deeper, more relevant insights, getting to the true heart of the customer experience and finding ways to improve it.
Sentiment Analysis Machine Learning
Sentiment analysis in machine learning is the process of using NLP and computational techniques to automatically identify and extract subjective information from text, such as opinions, attitudes, and emotions, and determine whether the overall sentiment of the text is positive, negative, or neutral. Machine learning is a subfield of AI that involves the development of algorithms and statistical models that enable computers to learn from data and make predictions or decisions without being explicitly programmed.
In the context of sentiment analysis, machine learning is used to build models that can automatically identify sentiment in text by learning from examples of labeled data. One of the main benefits of using machine learning for sentiment analysis is that it allows for more accurate and reliable sentiment analysis. Traditional rule-based approaches to sentiment analysis rely on handcrafted rules and heuristics to identify sentiment in text, which can be limited by the complexity and nuances of language.
By contrast, machine learning models and NLP algorithms can automatically learn to recognize complex patterns and relationships in the data, allowing for more accurate and robust sentiment analysis. Another benefit of using machine learning for sentiment analysis is that it can improve scalability and efficiency. This can help businesses save time and resources.
Natural language processing software plays a critical role in sentiment analysis by providing the tools and techniques needed to analyze and understand natural language text. Some of the key NLP techniques used in sentiment analysis include:
- Text preprocessing
- Part-of-speech tagging
- Named entity recognition
- Sentiment lexicons
By combining NLP with machine learning, organizations can build sophisticated sentiment analysis models that provide valuable insights into customer opinions, attitudes, and emotions. This can allow them to improve the customer experience and their business operations overall.
Sentiment Analysis Online
There are many different types of online sentiment analysis tools and platforms available, ranging from free, basic tools to more advanced, paid platforms that offer greater accuracy and functionality. It’s important to research sentiment analysis online tools in order to find the best solution for your business. Here are some of the most common types of sentiment analysis tools and platforms:
- Social media monitoring tools:
- Social media sentiment analysis tools are designed to monitor and analyze social media platforms to track mentions and sentiment around a particular brand, product, or topic. Hootsuite Insights is a good social media sentiment analysis example.
- Text analysis tools:
- These tools analyze text data from a variety of sources, including customer feedback forms, product reviews, and news articles. They use NLP techniques to identify and extract sentiment from text.
- Survey tools:
- Survey tools collect and analyze feedback from surveys, polls, and questionnaires. They use NLP and machine learning to identify sentiment and themes from the responses.
- Voice of the customer (VoC) platforms:
- These platforms are designed to capture and analyze customer feedback across multiple channels, such as email, chat, phone, and social media.
- Sentiment analysis APIs:
- These are programming interfaces that allow developers to integrate sentiment analysis capabilities into their applications or websites. They typically use machine learning algorithms to analyze text data and provide sentiment scores.
When choosing a sentiment analysis tool or platform, it’s important to consider factors such as accuracy, ease of use, pricing, and scalability. Some tools may be more suited to specific use cases, such as social media monitoring or customer feedback analysis, so it’s important to evaluate each option based on your specific needs and goals. It can also be helpful to view a sentiment analysis online demo to learn more about how a specific tool works.
How It Works
Gain a deeper level understanding of contact center conversations with AI solutions.
See a PreviewAggregate
Pull customer interaction data across vendors, products, and services into a single source of truth.
Analyze
Collect quantitative and qualitative information to understand patterns and uncover opportunities.
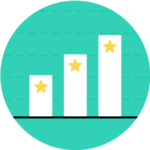
Activate
Confidently take action with insights that close the gap between your organization and your customers.
How Does Sentiment Analysis Work
So how does sentiment analysis work? Sentiment analysis analyzes and categorizes text data to determine sentiment or emotion within text. The process of sentiment analysis typically involves the following steps:
1. Text preprocessing: The first step in sentiment analysis is to preprocess the text data to remove any irrelevant information and prepare the text for analysis.
2. Sentiment scoring: Next, a sentiment score is assigned to each word or phrase in the text. This is typically done using a sentiment lexicon, a pre-compiled list of words and phrases with associated sentiment scores.
3. Aggregation: Once sentiment scores have been assigned to each word or phrase in the text, the next step is to aggregate the scores to determine an overall sentiment score for the text.
4. Post-processing: The final step is to perform post-processing on the sentiment analysis results to improve their accuracy and interpretability.
Viewing a sentiment analysis example is a great way to learn more about this type of software. For instance, a brand sentiment analysis example demonstrates how sentiment analysis can be used to analyze the sentiment surrounding a particular brand or product. Similarly, a sentiment analysis positive, negative neutral example shows how this software can be used to detect different types of emotions.
You might also view sentiment analysis example sentences to see what customers are specifically saying about your products or services. One sentiment analysis model might yield different results than another, so it’s important to understand different models and techniques. This will give you a better idea of how to use sentiment analysis to your advantage.
How To Do Sentiment Analysis
Learning how to do sentiment analysis is critical for any business that wants to better understand the customer’s voice and make changes to improve the overall customer experience. You can study a sentiment analysis example project to get a better understanding of how a company might use sentiment analysis to execute critical business initiatives.
For example, one organization might plan a project with the goal of analyzing the sentiment of customer reviews and generating insights to improve the customer experience. Working with a dataset of 1,000 customer reviews, the organization would begin by cleaning up the data and performing text analysis. Then, they would assign a sentiment score to each review based on the identified sentiment.
The next step in the project would be visualization and analysis, where the sentiment scores are visualized using charts and graphs to provide insights into the overall sentiment of the organization. Trends and patterns are identified and analyzed to identify areas for improvement. Based on the sentiment analysis results, the business is provided with actionable insights to improve customer experience and reputation.
By using a sentiment analysis tool online, you can carry out similar projects to better understand your customers, how they feel about your business, and what they are ultimately trying to tell you. The best sentiment analysis tools are those that provide detailed, actionable insights into customers’ experiences. They equip users with the information they need to address any issues or roadblocks in the customer journey and continually improve their offerings.