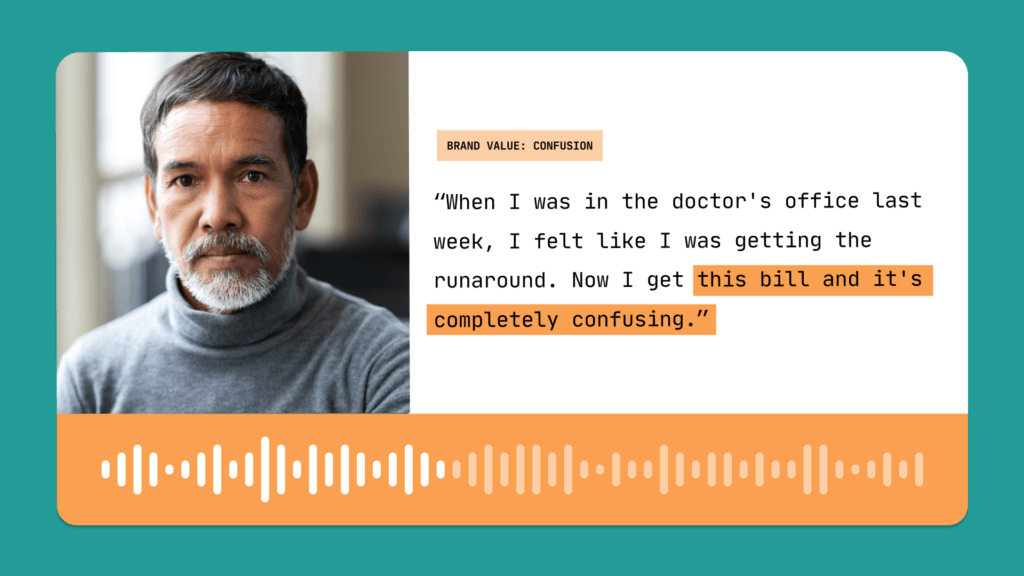
Insights that balance the head and the heart of CX.
Authenticx generates healthcare-specific NLU algorithms to share immersive and intelligent insights.
Learn MoreAI is changing the way healthcare listens. Authenticx analyzes customer conversations to uncover actionable insights.
Software that connects qualitative human emotion to quantitative metrics.
Collecting data is only part of the equation. Listen at scale to engage with the story your customers are sharing.
See Authenticx in Action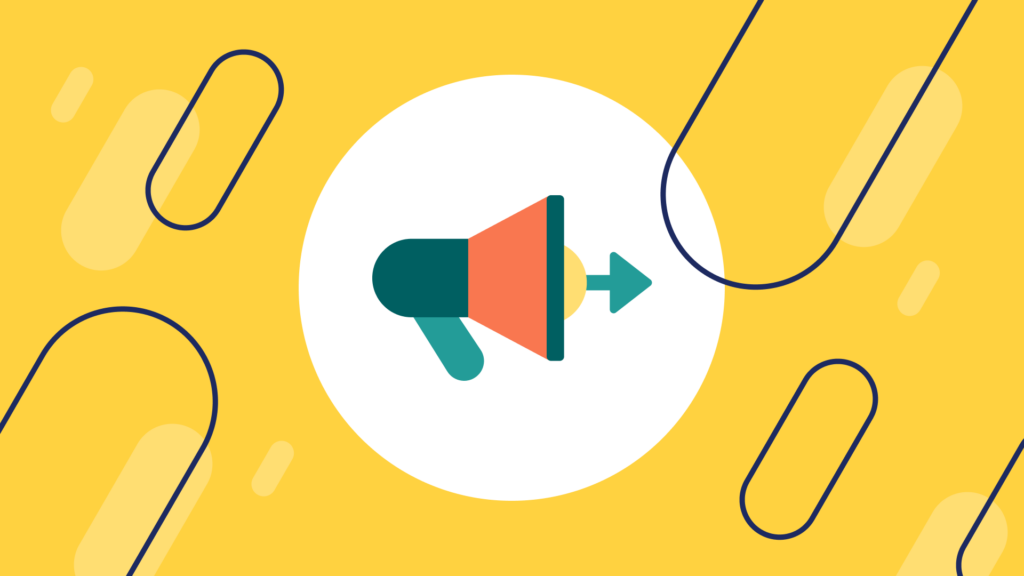
What Is Sentiment Analysis In Machine Learning
Sentiment analysis is the process of analyzing data to determine if it is positive, negative, or neutral. The process involves natural language processing based on textual data gathered from speech and text sources. The best model for sentiment analysis studies the subjective information expressed through opinions, appraisals, attitudes, and emotions, then classifies expressions as positive, negative, or neutral. Companies can benefit from understanding customer interactions at every touch point where textual data is captured. The challenge is how to do sentiment analysis. The process can be done using natural language processing and machine learning techniques. Machine learning techniques are gaining popularity because machine learning provides companies with a cost-effective way to analyze customer data quickly. Machine learning involves the use of computer systems developed to learn and adapt without the need for human interaction. When applying machine learning, computers can use algorithms and statistical models to analyze patterns in data resulting in drawing inferences from the data.
What is sentiment analysis in machine learning? Sentiment analysis using machine learning is a technique in which data is automatically analyzed to determine if customer interactions are positive, negative, or neutral. Sentiment analysis machine learning uses natural language processing to design the core building blocks of the data analysis process. Understanding how to do sentiment analysis is crucial for companies today. Companies set up a unique strategy for sentiment analysis, starting with data collection. Following data collection, a text cleaning occurs. For example, among all the text data captured, punctuation and stop words are not helpful in the sentiment analysis process, so they are removed during the cleaning process. The goal is to reduce words to their step before moving on to the analysis process. Following text cleaning, the pre-set algorithms can analyze the data to determine its sentiment or emotional meaning. At the end of the sentiment analysis process, companies can view the data and better understand customer interactions.
Machine learning in sentiment analysis is necessary due to the vast amount of data collected through texts, phone calls, reviews, and other methods. A comprehensive analysis of the data collected is almost impossible if attempted through manual processes. Machine learning adapts to the needs of individual users and is a scalable solution for data analysis. Sentiment analysis is vital for companies to elevate their sales and marketing efforts, adjust social media strategies and strengthen a consistent brand message. In addition, sentiment analysis can help companies conduct market research to gain an understanding of competitors.
How Does Sentiment Analysis Work
Sentiment analysis is something that happens in all interactions as people judge the meaning behind their interactions daily. A sentiment analysis machine learning code attempts to do the same thing, determine the sentiment, or feeling, behind textual interactions. The process of text sentiment analysis determines whether text gathered from social media posts, reviews, survey responses, text messages, emails, call center interactions, or any other form of text or captured verbal communication is either positive, negative, or neutral. In addition to positive, negative, or neutral, a sentiment analysis algorithm can be designed to determine if the text conveys happiness, anger, sadness, or amusement. Sentiment analysis can also help assess intent, such as interest, disinterest, or very interested.
Companies collect vast amounts of text data daily, so it is crucial to understand how sentiment analysis work. It is a subset of artificial intelligence (AI) and a form of natural language processing. Natural language processing is widespread and in use on many everyday platforms. For example, predictive text is a form of natural language processing. Another example includes chatbots, a feature many companies use as an online customer service tool. Natural language processing programs use different methods to simulate human interaction. Advanced algorithms are established to apply rule-based or automatic systems for analyzing text data from interactions. Data is collected and organized into sentiment analysis datasets. Rule-based sentiment analysis is established through manually defined rules to help determine what a sentiment analysis dataset means. Automated sentiment analysis uses deep learning models for sentiment analysis. Deep learning is a machine learning capability that directs computers to do or understand what comes naturally to humans. In relation to artificial intelligence, machine learning is a subset of artificial intelligence (AI), and deep learning is a subset of machine learning. Deep learning teaches filtering data through layers to predict and classify information. It is used to mimic the way the human brain filters information.
Sentiment Analysis Example
Sentiment analysis uses natural language processing techniques to understand opinions and feelings in customer feedback and interactions. Applying sentiment analysis empowers businesses to gain valuable insights about branding, products, and services. A popular sentiment analysis example includes social media monitoring. Through sentiment analysis, companies can mine social media data and extract underlying feelings through social media conversations, comments, and posts to understand how people are talking about a product or topic. Sentiment analysis example sentences show positive, negative, or neutral intent. For example, Twitter posts that tag a company and use verbiage such as “impossible to contact” or “excellent service” infer negative and positive sentiments, respectively. A negative neutral example may be “service was ok” or “ok service, but I won’t go back.” Applications of sentiment analysis in politics include predicting political outcomes. Algorithms are set using natural language processing and sentiment analysis to analyze people’s opinions and feelings about political topics and candidates.
Implementing machine learning sentiment analysis is a skill that must be learned to maximize the process’s benefits and design it to meet current business needs. A sentiment analysis example project is Amazon product reviews. Evaluating product reviews on Amazon is an excellent starting point for learning how to design and implement sentiment analysis techniques. Another sentiment analysis machine learning example includes tracking customer sentiment over time. Learning to track customer sentiment over time helps companies measure and understand when and why changes in customer sentiment occur. Algorithms drive the sentiment analysis process using a scoring method for words, voice inflections, and other factors to determine a customer’s overall feeling. For example, changes in speech rate, stress level as indicated by voice volume level, and frustration are evident in an interaction. Sentiment analysis is applied across many applications and scenarios for various reasons, such as using negative sentiments to improve services and using sentiment analysis of positive comments to promote businesses. Companies use sentiment analysis to monitor customer service departments or outsourced call centers and to monitor reviews and social media comments. Political candidates use sentiment analysis to monitor opinions about policy changes or campaign announcements. Sentiment analysis takes enormous amounts of customer-centered interaction data, removes unnecessary fluff, and delivers a thorough understanding of the people interacting with the brand, company, or entity.
How It Works
Gain a deeper level understanding of contact center conversations with AI solutions.
See a PreviewAggregate
Pull customer interaction data across vendors, products, and services into a single source of truth.
Analyze
Collect quantitative and qualitative information to understand patterns and uncover opportunities.
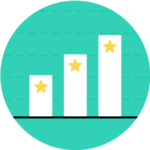
Activate
Confidently take action with insights that close the gap between your organization and your customers.
Sentiment Analysis Applications
Through sentiment analysis, businesses can categorize customer communication into positive, negative, and neutral categories. With this valuable information, companies can make well-informed decisions. Customer interactions occur across many channels, increasing the need for sentiment analysis tools. Sentiment analysis applications are helpful for social media monitoring, brand monitoring, customer support ticket analysis, customer service calls, product analysis, and market research. There are different types of sentiment analysis. For example, fine-grained sentiment analysis enables the analysis of each sentence part. In contrast, coarse-grained analysis is broader and applicable to an entire document or a whole sentence.
Applications of sentiment analysis in business often require a more detailed approach to sentiment analysis. Companies use aspect-based sentiment analysis to dig deeper into customer feedback by associating specific sentiments with defined product or service aspects. In this application, an aspect is a component or attribute of a product or service. For example, an aspect of a product may be the user experience of the product, and an aspect of customer service may be the company’s response time to a question or complaint. With aspect-based customer sentiment analysis, customer interactions are categorized into the aspects defined by the company. Examples of application of sentiment analysis in real life across various business sectors include brand reputation, improving services, and market research. Through sentiment analysis of positive, negative, and neutral analysis results, companies can take everyday real-life interactions such as social media comments and posts, online reviews, and customer phone calls and turn them into helpful insights.
Sentiment Analysis Models
Businesses have access to excessive amounts of data from customers today. Analysis of collected data helps companies make well-informed decisions about what works and what doesn’t. There are two types of data that help companies gain an in-depth understanding of customer interactions. Tangible data collected from surveys, feedback, reviews, and subjective data focused on feeling or the sentiment behind the responses.
Types of sentiment analysis include:
- Aspect-based sentiment analysis
- Fine-grained sentiment analysis
- Intent-based sentiment analysis
- Emotion detection
Sentiment analysis models are used to analyze and classify interactions within labels defined by the business. A sentiment analysis model is a way of customizing an analysis within a type. For example, when applying an emotion detection sentiment analysis, a company can define a model using specific verbiage such as “excellent service” or “it was ok.” Pre-trained sentiment analysis models use pre-determined words or phrases to analyze customer sentiment. The best algorithm for sentiment analysis is based on individual needs and company preferences. Sentiment analysis algorithm models include rule or lexicon-based models that rely on manually designed rules for classifying data and determining sentiment. Another model is an automated or machine learning model. With a hybrid sentiment analysis model, companies can benefit from both automatic and rules-based systems through automation and some flexible customization.