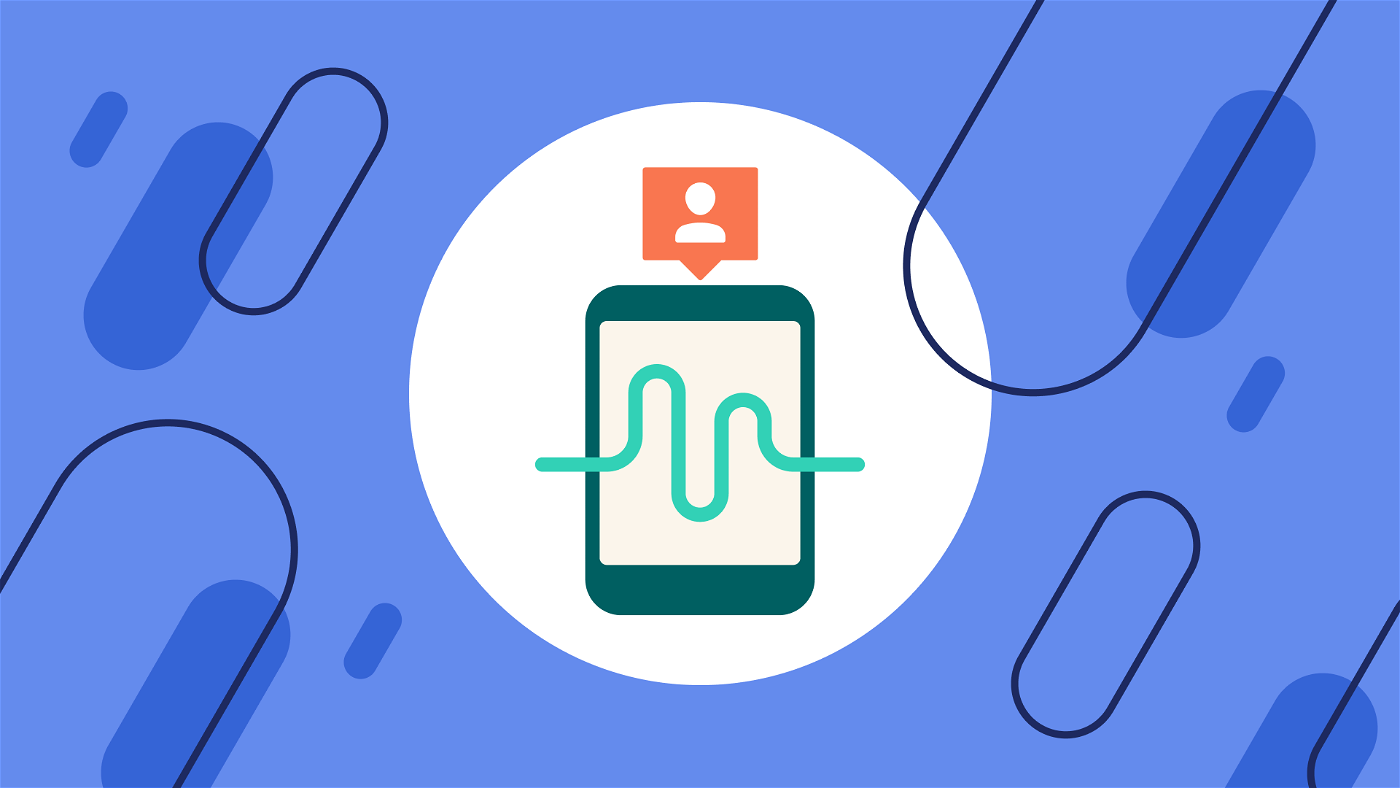
Humans and artificial intelligence (AI) go hand in hand.
Humans are genuine and emotional. Emotions are real and robust. AI is patterned and numeric. Numbers are analytical and precise.
But there isn’t a relation between artificial in the human.
But what if there is?
When humans hear “artificial intelligence”, feelings of hope, fear, and confusion could all be present simultaneously. That alone is a constructive irony in understanding why emotion still matters. It can’t be replaced when zoomed in and out at scale. In other words, emotions must be felt to drive action – and there are steps to moving from transactional to customer-centric by leveraging AI tools.
- Start with a plan (what, why).
- Move to understand personal expressions (who, where)
- End with acting on the data (how).
With forms of AI becoming more accessible to many people, there is a belief that understanding customers and patients can all be done through a digital bot, such as ChatGPT. In reality, this creates a compounded problem in communicating to understand each individual’s unique preferences. These types of AI bots bring in and release large amounts of unstructured information – information that lacks visibility and meaning that could create actionable insights that make sense of the collected data. Leveraging industry-specific (like healthcare) trained AI can produce the desired insights that are as sensitive and complex as each conversation – one that intentionally reads emotion and intelligently double-downs on human listening.
How AI Interacts with Humans and Technology
Unsurprisingly, when humans are introduced to artificial intelligence, there is immediate trepidation about how honest and real the data could be. To distribute a constructive and productive analysis – one that recognizes the potential of implicit bias as previously described – the data collected must be sprawling in both numbers and elements of diversity. If a customer-centric approach is desired, the ability to cover the population is only capable through the practical use of AI, that is, one weaved with human intervention. Both the human component of AI and machine learning must mutually benefit the process of actionable data analytics to improve the voice of the customer while increasing the status of care to the patient and the positivity of the employee.
Even when the balance of power between AI and humans can be continuously checked, the science behind AI interaction will be debated beyond surface-level and professional conversations. As outlined by NBC News, the concept of “responsibility” can be seen as subjective unless there is an industry (or positional) adoption of optimization. This is how breaking down deep learning becomes an integral part of the future of technology’s role in healthcare.
Loosely, deep learning is a technique within machine learning that builds an artificial neural network – in other words – it slowly teaches human representation. This is not a simple process to produce due to the impossibility of complete bias removal. As Michael Armstrong, Authenticx’s Chief Technology Officer detailed in Healthcare IT Today, “Algorithmic biases occur when designers use incomplete data lacking full representation of a specified patient population.” However, the recognition that data sets must be scrutinized for health inequities derived from biased data is the key to unlocking the potential of unbiased AI. Going further, deep learning might lend a hand to a more objective representation of human responses.
“…biases occur when designers use incomplete data lacking full representation… ”
Michael ArmstrongAs the machine learns, the more likely it is to create similar logic seen in human response to become a reliable tool for limiting bias in healthcare technologies. Once more, Michael Armstrong explains, “[deep learning] relies on more comprehensive and layered meanings through logical associations that match how human brains build neural networks of understanding, which limits the impact of individual biases in algorithmic results.” The negative results of a patient’s or customer’s health formed from bias can be restrained by being open to impartiality derived from training artificial intelligence to work with human-like thinking.
Understanding the Humans Served
While deep learning limits bias in data sets by understanding the human experience, Tech Monitor explains that context is what connects the human back to emotional AI. Humans are more likely to notice the atypical from the typical – this path is mirrored in how data analysis is better able to highlight action items to improve the customer experience. In the same way that a polygraph measures vital signs and movements, it cannot develop a human way of thinking (since different people react differently) by building a neural network, as aforementioned. This is spotlighted in healthcare doubly since most healthcare conversations are personal, as described by an article from AI Multiple. It is difficult for humans to comprehend the ins and outs of the healthcare-specific mentality to bring along each affected person, so why would it be any different for artificial intelligence to evaluate this? Trust and loyalty take time, this is a concept that not even machines can fast-forward – but according to a Forbes article, they can make it simpler when paired alongside human-to-human interactions. To dig deeper into understanding this relationship, studying the seven components of emotional intelligence (human-centered) can uncover links to unleashing deep learning and conversational intelligence that benefit the customer, the investor, and the company.
As explained in a Simplilearn article, emotional intelligence is composed of self-awareness, self-management, self-regulation, motivation, empathy, social skills, and relationship management. Taking a broader view, themes connecting these to one another are thought, recognition, and association.
- Thought: Develop the steps before acting by taking time to understand the whole conversation.
- Recognition: Develop perspectives and obstacles faced within conversations, such as social determinants of health.
- Association: Develop how potential outcomes can be directly impacted via if-then ideology in conversations.
By approaching AI through the human components of emotional intelligence, concepts such as deep learning can provide a more holistic representation that can verify or clarify conversational outcomes in healthcare with less bias present. No method is foolproof, but some do offer more benefits to listening at scale with the authenticity derived from unsolicited feedback.
Emotion Still Matters
When humans engage outside of their usual tendencies (at work, with family or friends, in a public setting such as dining out), they must intentionally maneuver the social setting to their comfort level while remaining open to sharing their own experiences and understanding others. According to an article from Harvard Business Review, people seek the pace and the balance of the unknown, so they often open more when they commit to it. How can people better grasp this balance necessary to grow and evolve? The answer lies in making emotion technical and measurable.
Emotional AI kicks down the barriers of comprehension and sentiment that get lost in the jumble of high volumes of conversational data withheld without analysis. When companies and organizations implement a process to utilize emotional AI (call center training, real-time feedback, operational decision-making, etc.), the voice of their employees and customers can provide actionable insights that drive impact.
According to IoT For All, there are three types of emotional AI: Text, audio, and video.
- Text, such as chat boxes or email, is used to teach the machine to learn the data as it collects more in quantity to improve its quality.
- Audio, such as calls, is used to measure the sentiment of both call parties via tone, speed, or pace. This can help ensure the caller has the correct number.
- Video, such as check-ins or virtual agent help, is used to analyze the context of the call better while opening training opportunities for agents.
Although the benefits of emotional AI in understanding the human are bountiful, a few arguments include inherent bias (machines learn what they are programmed to learn), standardizing emotional expression (different people react and show emotions differently), and discomfort of being monitored (although privacy can be strengthened, people feel vulnerable to digital oversight). But as explained, these arguments can be addressed and minimized through understanding the voice of the customer beyond the blanketed data.
One of the greatest ways to run a checks-and-balance line of thought is through authenticity. From the same Forbes article, ensuring diversity is present in the population makes the power of unsolicited feedback more authentic. Much like how genealogy companies collect identifiers through submissions – the more submissions, the more robust ability to correctly identify and update each subscriber’s genetic markers – the greater population specified in healthcare conversations, the more likely it is that the feedback and insights are real and actionable. According to an article in Internet Policy Review, since unsolicited data is fully conversational, it removes the presumption around emotional AI software being biased and supports the initiative of a culturally and socially diverse tool via deep learning concepts that develop continuous refinement. Subsequently, this can highlight simplified comparisons of cultures to better achieve a perspective that looks beyond a bias-based performance to craft one of the complexities of humans and humans in healthcare.
Are you ready to listen to your customers at scale?
Authenticx brings the human to healthcare through conversational intelligence. And we know we can help.
About Authenticx
Authenticx was founded to analyze and activate customer interaction data at scale. Why? We wanted to reveal transformational opportunities in healthcare. We are on a mission to help humans understand humans. With a combined 100+ years of leadership experience in pharma, payer, and healthcare organizations, we know first-hand the challenges and opportunities that our clients face because we’ve been in your shoes.
Want to learn more? Contact us!
Or connect with us on social! LinkedIn | Facebook | Twitter | Instagram | YouTube