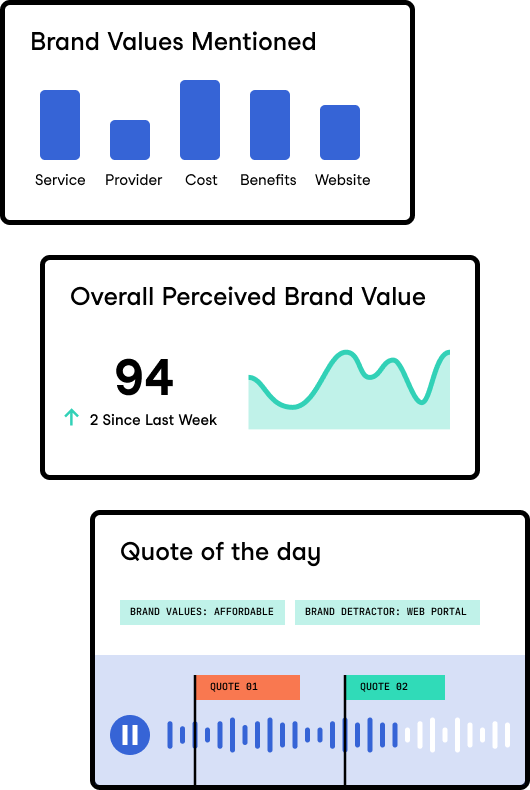
Extracting informational insights that speak to the head and the heart.
Authenticx generates NLU algorithms specifically for healthcare to share immersive and intelligent insights from unstructured text.
Learn MoreMyths vs. Facts: How Artificial Intelligence Is Changing the Way Healthcare Listens
Connect qualitative human emotion to quantitative metrics.
Data collection is only part of the equation. Listen and engage with the story your customers are sharing.
See Authenticx in Action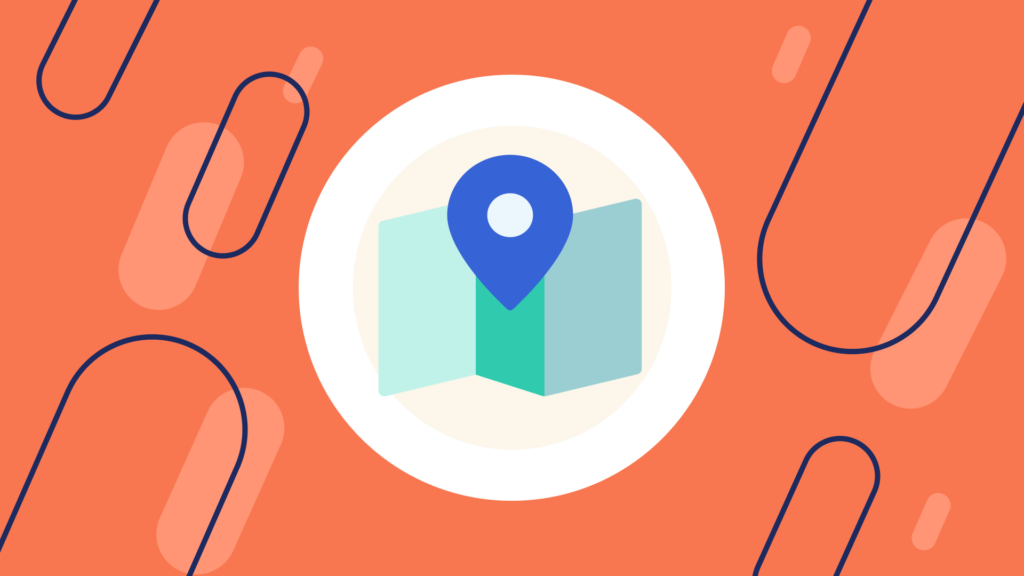
Extracting Information From Unstructured Text Using Algorithms
Unstructured data, such as open-ended survey responses, social media posts, and customer feedback, can be challenging to analyze and interpret due to its unorganized nature. However, with the help of machine learning algorithms and natural language processing techniques, organizations can now extract valuable insights from unstructured data. Extracting information from unstructured text using algorithms allows companies to analyze large amounts of text-based data quickly and accurately. This can, in turn, help them to make data-driven decisions.
AI algorithms can categorize and classify unstructured data into meaningful themes and topics, which can be used to identify trends, patterns, and insights. Sentiment analysis algorithms can also be used to analyze the sentiment expressed in the text, helping organizations understand the emotional state of their customers or employees.
The use of AI algorithms to extract facts from text is changing the way organizations gather, analyze, and interpret survey data. It allows businesses to gain a deeper understanding of customer needs, preferences, and opinions, which can be useful for improving products and services. The insights derived from unstructured data analysis can also be used to identify potential problems, develop new strategies, and make more informed business decisions.
Text mining applications can be used in a wide range of fields. In business, text mining enables customer feedback analysis, sentiment analysis, and brand reputation management. By analyzing customer feedback and reviews, businesses can identify areas for improvement and enhance their products and services to meet customer needs. Sentiment analysis can also help organizations to understand how customers feel about their brand and make decisions accordingly.
This technology can also be used in social media for opinion mining and trend analysis. Companies can identify what people are talking about and what topics are trending. This information can be used for marketing, product development, and customer engagement. Those in education might use text mining for plagiarism detection and language learning. What’s more, language learning applications help users identify areas where learners need more practice and provide personalized feedback.
In healthcare, text mining can be used for clinical decision-making and medical research. Analyzing medical records allows doctors to identify patterns and make more accurate diagnoses. Additionally, with text mining, researchers can identify new drug targets and study the effectiveness of different treatments.
Authenticx’s call center Speech Analyticx software combines qualitative and quantitative data to help healthcare organizations understand and engage with the stories patients are telling. This can lead to better outcomes for all involved.
Deep Learning For Specific Information Extraction From Unstructured Texts
Extracting information from unstructured text using algorithms is called text mining, and one of the most powerful techniques in text mining is deep learning. Deep learning for specific information extraction from unstructured texts involves training artificial neural networks to automatically learn and recognize patterns and relationships between words and phrases. This allows deep learning algorithms to perform tasks such as named entity recognition, part-of-speech tagging, sentiment analysis, and text classification with high accuracy and efficiency.
As a result, deep learning has become a key AI term for extracting information from unstructured text using algorithms. It has applications in a wide range of fields, including business, healthcare, and education. The benefits of extracting information from unstructured text using algorithms in AI include:
- Scalability: Algorithms can process large volumes of unstructured text data much faster and more accurately than humans can, making it possible to extract valuable insights from large datasets.
- Consistency: Algorithms are not subject to human biases or errors, so the results of text mining are more consistent and reliable than those obtained through manual analysis.
- Accuracy: AI-based algorithms can identify patterns and relationships that humans may miss, leading to more accurate and comprehensive insights.
- Cost-effectiveness: Text mining algorithms automate tasks that would otherwise require significant time and resources to perform manually, making it a more cost-effective solution for information extraction.
- Customization: AI algorithms can be trained to recognize specific features and patterns of interest. This makes it possible to extract information that is tailored to specific business needs or research questions.
- Innovation: By leveraging the power of AI for information extraction from unstructured text, businesses and researchers can uncover new insights, develop innovative products and services, and make more informed decisions.
Information Extraction NLP Example
Natural Language Processing (NLP) is a branch of AI that focuses on enabling machines to understand and process human language. One of the most common applications of this technology is information extraction from text NLP. It involves automatically identifying and extracting relevant information from unstructured text data, such as social media posts, customer reviews, or news articles.
NLP can be used for information extraction in a variety of ways. For example, it can identify entities within a text document and classify them according to their type. It can also be used to extract relationships between entities, such as who works for which company, or which products are associated with certain brands. Another common use case for NLP is extracting events or actions described in a text.
A good information extraction NLP example is sentiment analysis, which involves analyzing a text to determine the writer’s attitude or opinion toward a particular topic. Sentiment analysis is often used by businesses to monitor customer feedback and assess product or service quality. Another information extraction example is named entity recognition (NER), which identifies and classifies entities mentioned in a text. For instance, a company might use NER to extract customer names and addresses from emails or social media posts for targeted marketing campaigns.
It can be helpful to view an information extraction NLP tutorial when getting started with this technology. Here’s a step-by-step example to follow:
- Define the problem and gather data: The first step in any information extraction project is to define the problem you want to solve and gather the necessary data.
- Preprocess the text: The next step is to preprocess the text data to prepare it for analysis. This might include tasks such as removing stop words, tokenizing the text, and performing part-of-speech tagging.
- Define the entities to extract: In this step, you’ll define the entities you want to extract from the text. For example, you might want to extract all the company names that appear in the text.
- Train the NER model: If you’re using machine learning algorithms for NER, you’ll need to train a model on a labeled dataset. This process involves annotating the text data with the entities you want to extract and using that data to train a machine-learning model.
- Extract the entities: Once you’ve trained your NER model, you can use it to extract the entities from the text. You can apply the model to new text data and it will automatically identify and classify entities based on the patterns it learned during training.
- Analyze the extracted entities: The final step is to analyze the extracted entities to gain insights into your data. For example, you might want to count the number of times each company name appears in the text, or analyze the sentiment associated with each company.
How It Works
Gain a deeper level understanding of contact center conversations with AI solutions.
See a PreviewAggregate
Pull customer interaction data across vendors, products, and services into a single source of truth.
Analyze
Collect quantitative and qualitative information to understand patterns and uncover opportunities.
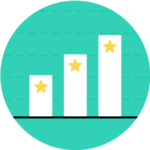
Activate
Confidently take action with insights that close the gap between your organization and your customers.
Information Extraction Survey
An information extraction survey is a type of survey designed to gather data on specific pieces of information from a set of text documents. When learning how to extract meaning from text, it’s important to know what you’re looking for. Intelligent tools can help with this process, but it’s always a good idea to know what you’re looking for.
Extracting information from unstructured text using algorithms is called natural language processing and can be extremely helpful when it comes to interpreting and analyzing surveys. It can also be useful if you are looking to extract an address from text in machine learning.
Text Mining vs. NLP
Text mining and NLP are related fields, but they have different focuses and techniques. It involves the use of statistical and machine learning techniques to extract useful information from large collections of text data. This data can be structured or unstructured, and text mining techniques can include tasks such as topic modeling, clustering, and entity extraction.
NLP, on the other hand, is a more specialized field that focuses specifically on the interaction between computers and natural language. NLP techniques involve the use of algorithms and statistical models to analyze and understand human language, including tasks such as sentiment analysis, part-of-speech tagging, and named entity recognition.
Understanding text mining vs NLP is critical for anyone working with information extraction tools. Viewing text mining examples is a great way to learn more about how the text mining process differs from NLP. For instance, text mining in R is the process of using the R programming language and associated packages to analyze and extract useful information from text data. There are a number of tasks that can be performed this way, including text visualization and sentiment analysis.
It’s also important to compare text mining vs. text analytics and understand the differences between the two. Text mining, also known as text data mining, is the process of discovering useful information from large collections of unstructured text data. Text analytics, on the other hand, is a broader term that encompasses a range of techniques for analyzing and extracting insights from text.
Through technologies like text mining and NLP, organizations can obtain deeper insights into their data, and ultimately boost revenue by improving business outcomes.