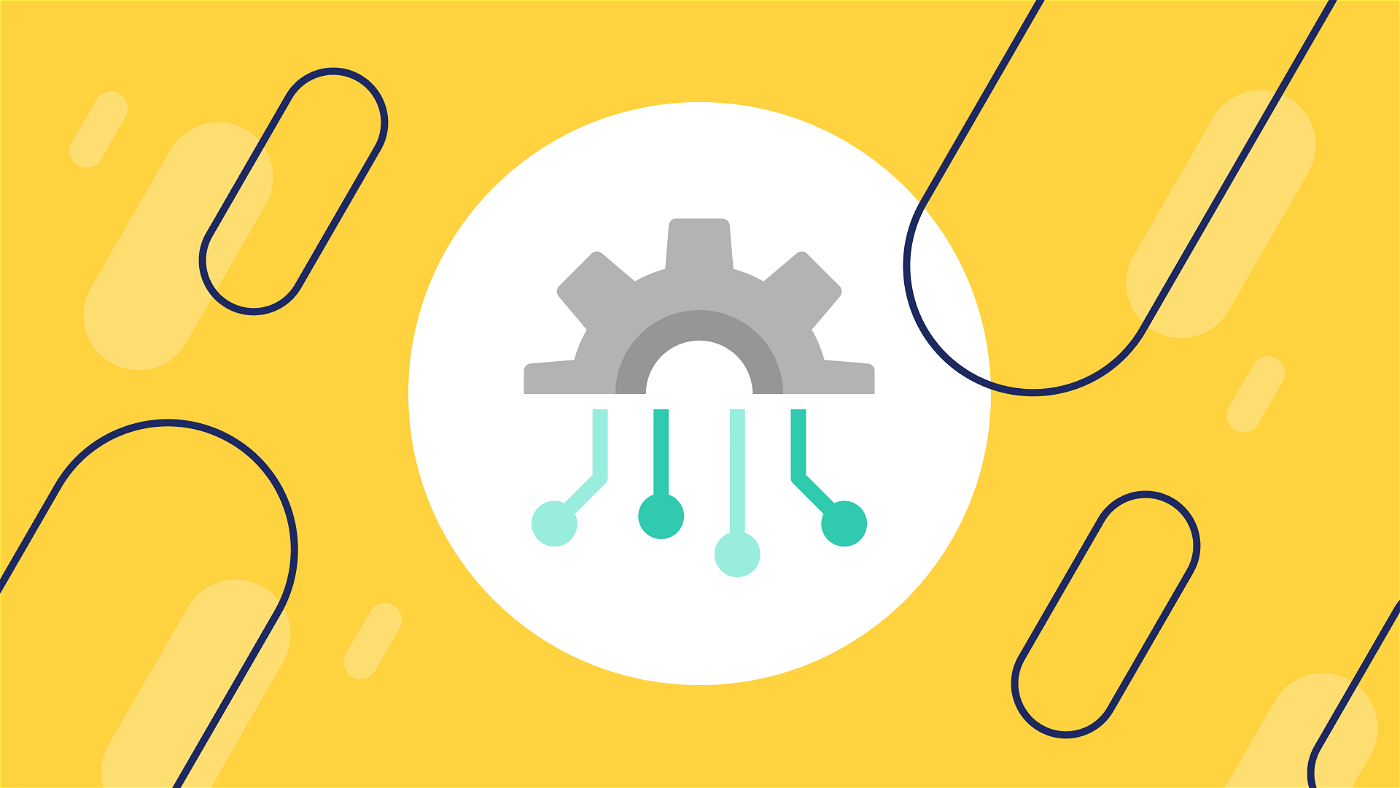
Authenticx’s VP of Product Innovation Michael Armstrong and CEO Amy Brown recently sat down to discuss a new concept they’re calling accessible machine learning and its potential impact on the marketplace.
Michael, you’ve recently talked about a concept you call accessible machine learning. What do you mean by that?
Michael: I guess I said this the other day in a meeting and Amy was like, “What was that thing you said?” I didn’t even realize I said it, but once she pointed it out, I started thinking about it. It’s a concept I’ve been thinking about for quite some time and that is that machines can do a lot and they can tell us a lot, but they can’t tell us everything or do everything.
To suggest that AI can do everything that a user or analyst can, it’s just not possible right now. We don’t have that level of sophistication. Quite frankly, I have a hard time understanding other humans, let alone building a machine that would understand other humans. So that’s sort of some background around this idea of accessible machine learning.
The way I think about it is this (and really this isn’t groundbreaking), but I want to give people tools, – access to the output of a model. Maybe I have some neural network-based model that I built that gives me output and I want users to see that output. I want them to see what the machine’s telling them and then I want them to be able to provide this feedback loop back into the machine to say, “Hey this does work,” or “This doesn’t work.” And again, it’s not really groundbreaking, I just don’t see it happening in the marketplace.
So we don’t just want to provide a score or output. We want to build a system that is continually getting better and giving our clients better insights, more effective, more efficient insights, and we want the machine to interact with the analyst so that there’s more of a feedback loop.
I want analysts to have tools that we use, that a data scientist might use, without having to know the data prep. I don’t want them to have to know about the data prep or various underlying statistical techniques, but I want them to be able to query the data, query the machine in a way that’s natural to them, that’s a part of their workflow, that’s part of their process.
Amy: I’d love to take a stab at what accessible machine learning means to me based on my understanding of Michael’s vision.
What it means to me is you have non-technical users who are adding value to their business place by using our platform to tag and label components of conversations that turn into insights that can be used immediately by the organization. All the while, they don’t have to realize that their tagging and labeling is improving the model for improvement in automated insights finding.
So it’s almost like leveraging a very non-technical data source to more efficiently, more effectively, more powerfully do their job, and also tackling machine learning and improvements within machine learning over time. Those two things have not been put together, from my view of the marketplace.
It’s like if a company wants to take on a machine learning effort, they do what Michael suggested, which is a plan where they consume tons and tons of data over months and months and waiting. The rest of the business, the business that’s relying on insights from the data to do something with, they’re waiting.
Whereas what we’re doing is we’re taking a resource that already exists within the enterprise – business analysts, compliance analysts, quality analysts, customer experience analysts. We’re giving them tools to more effectively consume one of the richest data sources that they’re not using today.
Today they’re sifting through surveys. Today they’re sifting through online reviews. These one-directional feedback sources where it’s a score and maybe a line of text and maybe why they didn’t like their customer experience. We’re giving these analysts a bi-directional conversation of unsolicited customer input and feedback that’s coming from their own words.
They’re able to use that data, analyze it, tag it, label it, turn it into call montages, have visualizations. All the while (so, immediate value here) we’re preparing models for greater and greater automation and reliance on AI to do better and better. And to prepare those analysts with pre-determined insights by a machine. So it’s certainly not an easy problem to solve and it’s certainly not a quick one. This is not an auto-magical you turn on the switch, but it’s the right one and it’s a more honest approach at getting real value from this data.
You can listen to Amy and Michael’s entire conversation here.