Your customers are talking. Are you listening?
Leverage unstructured data sources to answer questions, provide quality service, and enhance customer support with artificial intelligence, machine learning, and natural language processing.
Start Listening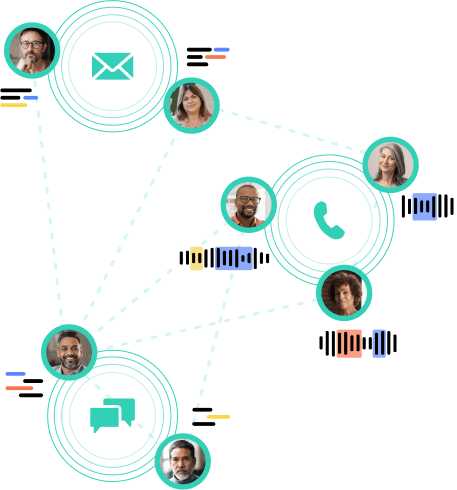
Aggregate topics, themes, and patterns from millions of conversations to drive relevant and impactful insights. Learn how AI listens at scale.
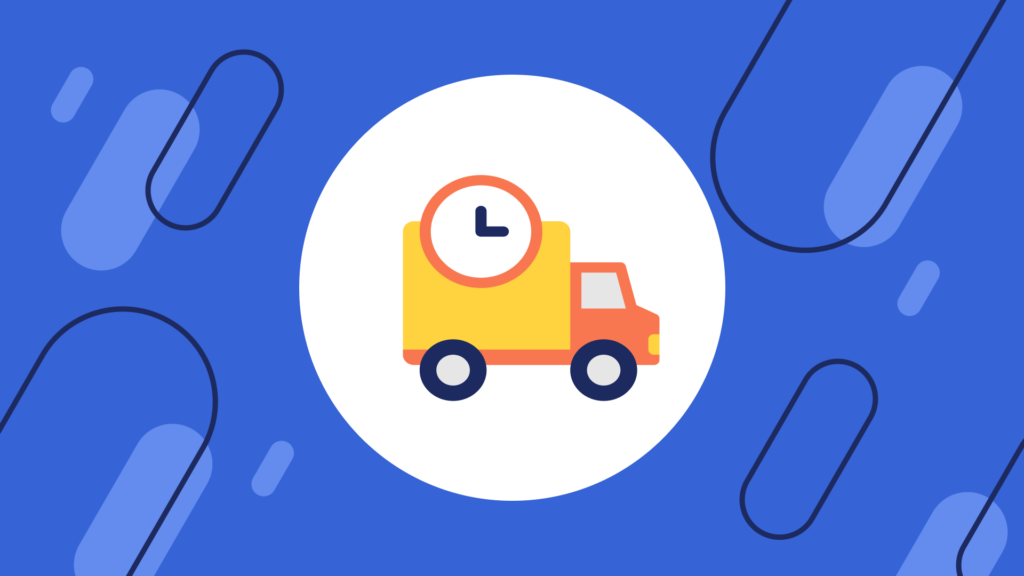
Preventing Refill Delays Amid Supply Chain Disruption
Using Authenticx AI, a life sciences manufacturer was able to prevent customer confusion and concern, while instilling confidence in their program during a potential labor strike.
Generative AI in Healthcare
Generative AI has emerged as a transformative technology within the healthcare sector that is poised to redefine the way organizations approach diagnosis, treatment, drug discovery, and patient care.
Generative AI in healthcare leverages complex algorithms to create new data based on patterns and information learned from existing datasets. This capability holds immense promise in areas such as medical imaging, where AI can enhance the accuracy of diagnoses by generating high-resolution images from limited data. Moreover, generative AI in medicine makes it possible to develop personalized treatment plans by predicting patient responses to various therapies.
Generative AI in the News
AI news frequently showcases breakthroughs in generative AI medicine. For example, by generating novel molecular compounds, AI can help accelerate the drug development process and potentially lead to faster identification of new treatments for diseases. This not only expedites the time-to-market for drugs but also reduces costs associated with traditional trial-and-error methods.
Generative AI Healthcare Applications
However, the use of generative AI in healthcare extends beyond diagnostics and drug development. Medical professionals can also use it to synthesize patient data for training. This allows them to practice their skills and decision-making in risk-free environments. Additionally, generative AI can contribute to the design of prosthetics and medical devices by enabling personalized solutions that match the unique anatomy of each patient.
Challenges of Generative AI in Healthcare
Despite its many promising applications, the introduction of generative AI in medicine is not devoid of challenges. Data privacy, ethical considerations, and regulatory compliance remain critical concerns for healthcare providers. Striking a balance between innovation and patient safety will be paramount as the medical industry moves forward with generative AI.
As new developments continue to break ground, it becomes increasingly evident that generative AI holds the potential to reshape the future of medicine and healthcare. From accurate diagnostics and personalized treatments to drug discovery and medical education, generative AI is already revolutionizing the way healthcare is practiced and researched.
What Is Generative AI?
Generative AI, also known as Generative Artificial Intelligence, is a specialized subset of AI technology designed to produce creative or innovative outputs. In contrast to conventional AI methods that adhere to predefined rules, generative AI learns from existing data and generates fresh outputs resembling learned patterns. This enables it to create a broad spectrum of human-like content, including text, images, music, and more.
In healthcare, the integration of generative AI holds transformative potential. Healthcare providers began exploring the prospects of AI in the early 2000s. However, it was the rise of deep learning, particularly neural networks, that catapulted generative AI into the healthcare spotlight.
As deep learning gained traction, generative adversarial networks (GANs) emerged, marking a pivotal juncture in generative AI’s integration into healthcare. GANs introduced a paradigm where two neural networks — a generator and a discriminator — engage in a competitive interplay to produce authentic data. This innovation formed the cornerstone of generative AI’s role in healthcare.
The potential of generative AI, particularly in medical imaging, has since captured healthcare providers’ attention. Generative AI models trained on extensive medical image datasets are capable of crafting high-fidelity synthetic images that hold value for diagnostics, surgical planning, education, and more. For example, ChatGPT healthcare prompts entered and screened by medical providers could aid in clinical decision-making by generating patient-specific insights.
Over the years, the impact of generative AI has also extended to drug discovery and personalized medicine. Researchers have found some success in leveraging generative AI to propose novel molecular structures and predict patient treatment responses.
Generative AI vs. Machine Learning
Generative AI is different from traditional machine learning. Generative AI learns from unlabeled datasets to generate novel outputs, whereas machine learning simply classifies or predicts based on existing labeled data.
Generative AI In Healthcare Examples
Here are some compelling examples that showcase the diverse ways generative AI is being harnessed in the healthcare industry:
Generative AI in Clinical Trials
Generative AI shows promise in optimizing clinical trial design by helping providers simulate various patient scenarios and analyze different possible treatment outcomes. This capability can expedite trial planning and ultimately lead to more efficient and cost-effective trials.
Generative AI in Drug Discovery
Generative AI can also significantly accelerate the process of identifying potential drug candidates by generating novel molecular structures and predicting their properties. This can reduce the time and resources needed for drug development.
Generative AI in Medical Imaging
Generative AI can also generate synthetic images that aid healthcare providers in diagnosis and treatment planning. For instance, generative AI can use limited data to create high-resolution images that allow healthcare providers to detect anomalies more accurately.
Generative AI in Life Sciences
Generative AI has the capacity to revolutionize the study of biological systems by simulating intricate biochemical interactions and genetic pathways. This ability allows generative AI to offer insights into disease mechanisms, potentially paving the way for the discovery of new treatment targets.
Generative AI in Healthcare Examples
Generative AI’s potential extends further still; healthcare providers can develop personalized treatment plans by using generative AI to predict patients’ physical responses to various therapies. Generative AI can also aid in the design of customized prosthetics and medical devices that are based on specific patient anatomies.
These are just a few of the exciting applications of generative AI in healthcare. Examples like these will likely continue to shape the healthcare industry for years to come.
How It Works
Gain a deeper level understanding of contact center conversations with AI solutions.
Learn MoreAggregate
Pull customer interaction data across vendors, products, and services into a single source of truth.
Analyze
Collect quantitative and qualitative information to understand patterns and uncover opportunities.
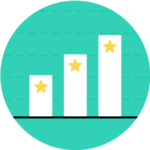
Activate
Confidently take action with insights that close the gap between your organization and your customers.
Generative AI Risks
While generative AI holds immense promise in the healthcare industry, it also presents certain risks that healthcare providers must navigate carefully.
Generative AI and HIPAA Compliance
One of the primary concerns with generative AI in healthcare is compliance with regulations such as the Health Insurance Portability and Accountability Act (HIPAA). The use of AI in healthcare typically involves sensitive patient data, and any mishandling or breach of this data could result in severe penalties for the healthcare organization.
Unintended Bias and Misinterpretation
Generative AI models learn from large datasets, which might contain biases. This could lead to the creation of biased outputs or misinterpretation of information, potentially influencing medical decisions and exacerbating healthcare disparities.
Limited Explainability
Generative AI models often lack transparency. The “black-box” nature of these models can be problematic when it comes to justifying medical decisions, which are typically required to be explained in detail for ethical and legal reasons.
Generative AI Lawsuit Risks
The use of generative AI in healthcare could create legal complications if a generated output results in medical errors. Providers could face lawsuits if they rely solely on AI-generated insights without proper human oversight.
Ethical Considerations
Generative AI’s contributions to medical decision-making have also given rise to numerous ethical dilemmas. Many healthcare professionals feel that generative AI is not yet ready to responsibly participate in the provision of medical care. This concern is the driving force behind innovative generative AI projects like Hippocratic AI, a generative AI developed specifically with the ethical implications of healthcare decision-making in mind.
Data Security
Generative AI models require large datasets for training. The storage of these datasets by healthcare organizations necessitates robust data security measures to protect against cyber threats and prevent unauthorized access to patient information.
As generative AI continues to play an important role in the healthcare industry, healthcare providers must remain vigilant in addressing risks like these to ensure patient safety and privacy and maintain compliance with essential regulations.
The Future of AI in Healthcare
Generative AI looks set to play a central role in the future of healthcare. While no one knows for sure exactly what to expect, the advancements enabled by generative AI hold the potential to usher in a new era of personalized, efficient, and innovative medical practices.
AI in Healthcare: 2023
In 2023 and beyond, healthcare professionals can expect to witness the continued integration of generative AI across various domains.
In medical imaging, generative AI models will likely further enhance the ability to generate high-resolution images from limited data, enabling more accurate and timely diagnosis. This could revolutionize the way radiologists and other healthcare professionals interpret medical images.
Generative AI in healthcare is also likely to fuel breakthroughs in the discovery and development of new drugs. By simulating molecular structures and predicting their properties, AI-driven models are capable of accelerating the identification of novel drug candidates. This ability could significantly reduce the time and resources required to develop new drugs.
The future of AI in healthcare is also likely to witness the rise of innovative generative AI healthcare startups. Generative AI startups in the healthcare industry will be able to collaborate with healthcare providers to address complex challenges, from predicting patient responses to designing tailored medical devices. These startups will leverage AI’s creative potential to develop cutting-edge solutions for personalized medicine, patient engagement, and more.
Furthermore, the convergence of generative AI with other technologies like Internet of Things (IoT) and wearable devices will enable real-time monitoring, paving the way for more proactive healthcare interventions. Patient-specific insights generated by AI models can empower clinicians to deliver individualized treatments and interventions that simultaneously improve patient outcomes and reduce healthcare costs.
With a growing ecosystem of startups driving continuous technological innovation, the future of AI in healthcare looks undeniably promising. Generative AI startups may be set to transform the way healthcare is delivered and experienced for many years to come.