Healthcare is changing and the stakes are higher.
Healthcare conversations are different, the system is complicated, and compliance is critical. Understand the 5 ways healthcare data analysis is changing.
Read How Healthcare Data is Changing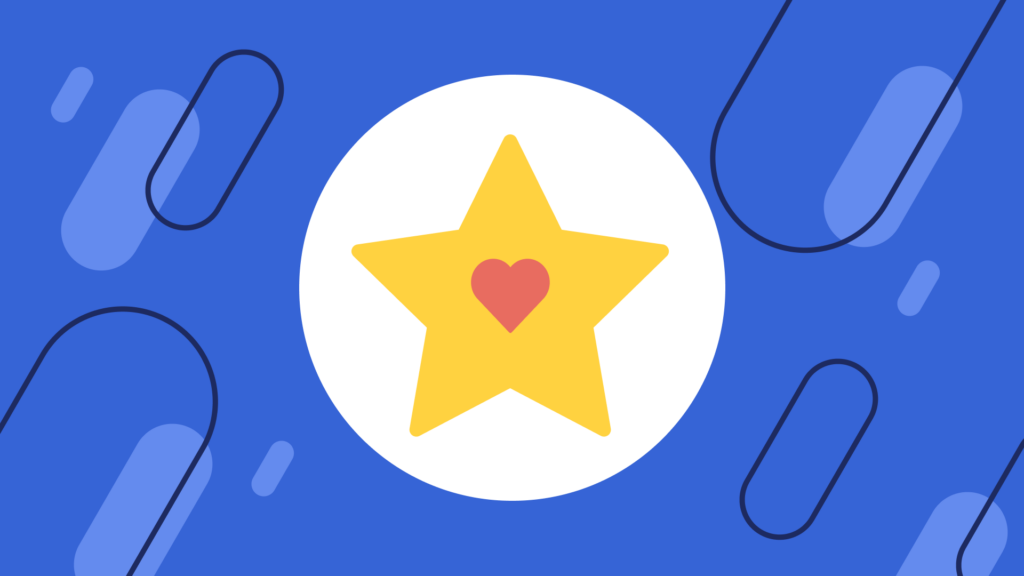
Learn how AI is unlocking insights across healthcare as we debunk 5 common myths around this emerging tech.
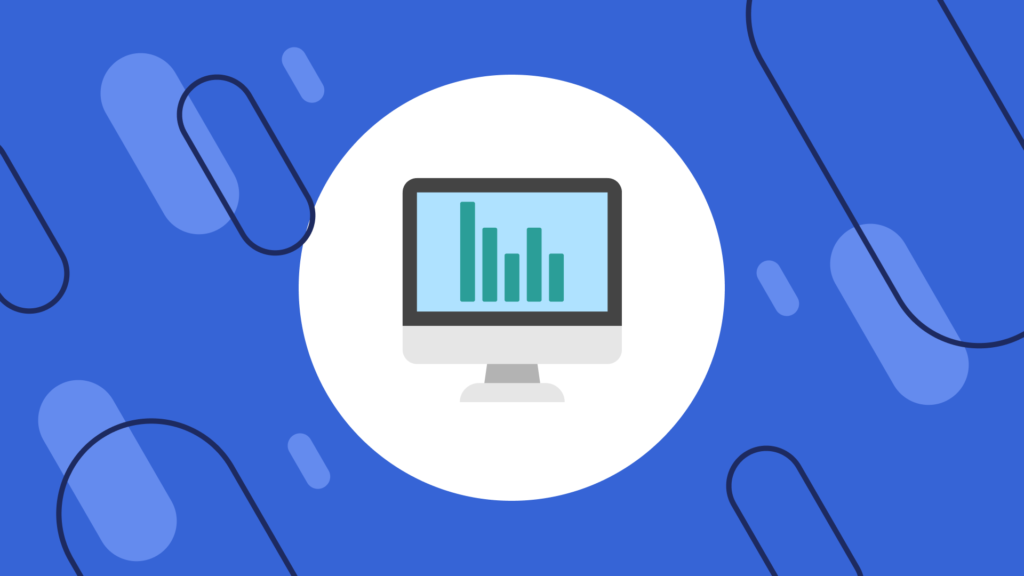
Increase monitoring and reduce compliance observations
Centralize and streamline compliance monitoring to flag high-risk events, escalate critical errors, and get context around driving risk factors.
See Authenticx in ActionPredictive Analytics Call Center
Predictive analytics in the context of call centers utilizes advanced algorithms to analyze historical call center data and derive insights that enable businesses to anticipate future trends, optimize resources, and improve performance. By harnessing the power of predictive analytics in a call center, companies can streamline their operations and improve customer satisfaction.
Call center data analytics forms the backbone of predictive analytics for call center operations, as it involves the collection and analysis of vast amounts of data. This data, often referred to as the call center dataset, may encompass call durations, call volumes, customer demographics, call resolutions, agent performance metrics, and customer feedback.
Call center analytics software is a crucial component of predictive analytics because it offers a sophisticated platform for data processing, visualization, and modeling. These software solutions are designed to handle the complexities of call center data and provide actionable insights that managers and decision-makers can rely on.
Likewise, a call center analytics dashboard is an essential tool that offers a comprehensive, real-time view of KPIs and other relevant metrics in an easy-to-understand visual format. The dashboard allows managers and supervisors to monitor the effectiveness of their call center operations, track the progress of their teams, and identify areas that require attention or improvement.
Predictive analytics for call centers can be applied in the following ways to improve performance and efficiency:
- Workforce management: Predictive analytics can forecast call volumes and average handling times, enabling managers to optimize workforce scheduling. This helps to ensure that there are sufficient agents available to handle the expected workload while minimizing idle time and overtime costs.
- Quality assurance: By analyzing historical data on agent performance and customer feedback, predictive analytics can be used to identify areas where additional training or coaching may be necessary, thereby improving the overall quality of service.
- Customer segmentation: Analyzing customer demographics and interaction data can help call centers identify specific customer segments and tailor their communication strategies accordingly, resulting in more effective and personalized interactions.
- Churn prediction: Centers that analyze customer interaction data can more easily identify the early warning signs of potential customer churn. This enables them to take proactive steps to retain these customers and reduce attrition rates.
- Real-time decision-making: By integrating predictive analytics into the call center analytics dashboard, managers and supervisors can make data-driven decisions in real-time, ensuring that their teams are always operating at peak efficiency and delivering exceptional customer experiences.
Types of Predictive Analytics
Call centers have access to various types of predictive analytics, each designed to optimize different aspects of their operations. Speech analytics, text analytics, and data analytics are three key approaches that can significantly enhance call center performance prediction and provide actionable insights for continuous improvement.
Speech analytics focuses on analyzing the audio of customer-agent interactions. It employs advanced algorithms to detect patterns, emotions, and keywords in conversations. By identifying potential issues, speech analytics offers call center predictive solutions that help streamline processes and pinpoint areas for agent training.
Text analytics, on the other hand, deals with the analysis of written communication, such as emails and chat transcripts. It extracts valuable insights from unstructured text data by highlighting patterns and sentiment. Text analytics can be instrumental in enhancing customer support, ensuring timely responses, and personalizing customer interactions.
Data analytics encompasses the analysis of structured data. It helps identify correlations and trends in data to drive decision-making and improve call center efficiency. Data analytics forms the foundation of call center predictive modeling, enabling businesses to forecast future trends and optimize resources.
Predictive analytics questions and answers can be addressed using these three approaches, providing businesses with a holistic view of their call center operations. Some call center predictive solutions that can be derived from these approaches include:
- Sentiment analysis: Speech and text analytics can gauge the emotions and sentiments of customers during interactions.
- Keyword extraction: Both speech and text analytics can detect critical keywords and phrases used by customers.
- Agent performance analysis: Data analytics can evaluate agent performance metrics, such as average handling time and first-call resolution.
- Workload forecasting: By analyzing call volumes and durations, data analytics can predict future workloads.
- Churn prediction: By combining insights from all three analytics types, call centers can develop models to predict customer churn.
Application of Predictive Analytics
Predictive analytics for call centers involves the application of predictive analytics to forecast future trends, optimize resources, and improve performance. These techniques empower businesses to make data-driven decisions, enhance customer experiences, and streamline operations.
Call center analytics use cases cover various aspects of operations, including customer segmentation and churn prediction. The application of predictive analytics in call centers can lead to significant improvements in efficiency and customer satisfaction. This, in turn, helps promote business growth and boost profitability.
Different use cases call for different approaches, and there are several predictive analytics techniques used in call centers today. They include machine learning algorithms, statistical models, and data mining approaches. These techniques analyze historical data to identify patterns and correlations and forecast future trends.
Call center forecasting with analytics is a crucial application that helps businesses anticipate call volumes, average handling times, and workload distribution. By leveraging predictive analytics, call centers can optimize staffing and scheduling. This helps ensure that enough agents are available during peak periods while minimizing idle time and overtime costs.
Emphasizing predictive analytics call center optimization is key to improving center operations in general. By analyzing various performance metrics, businesses can identify areas that require improvement and implement targeted solutions. Very often, this requires agent training, process, and technology upgrades.
Predictive analytics for call centers can also be used to segment customers based on demographics, interaction history, and preferences. This segmentation enables call centers to deliver personalized experiences, improving customer satisfaction and fostering long-term loyalty.
Churn prediction is another essential application that helps businesses identify customers at risk of leaving. Analyzing interaction data and customer behavior, predictive analytics can be used to detect early warning signs of potential churn so that centers can take proactive steps to retain these customers and reduce attrition rates.
How It Works
Gain a deeper level understanding of contact center conversations with AI solutions.
Learn MoreAggregate
Pull customer interaction data across vendors, products, and services into a single source of truth.
Analyze
Collect quantitative and qualitative information to understand patterns and uncover opportunities.
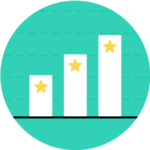
Activate
Confidently take action with insights that close the gap between your organization and your customers.
Predictive Analytics Examples
Predictive analytics examples in call centers demonstrate the power of data-driven decision-making and its impact on operational efficiency and customer satisfaction. The following predictive analytics case study examples highlight the diverse ways in which businesses have leveraged analytics to improve their call center operations.
Consider a large telecommunications company that implemented a combination of descriptive, predictive, and prescriptive analytics to optimize its call center workforce management. The company used historical data on call volumes, durations, and agent availability to forecast future workload patterns.
Ultimately, using predictive analytics, they were able to adjust agent scheduling, reduce wait times, and minimize idle time. As a result, the company saw a significant reduction in overtime costs and a noticeable improvement in customer satisfaction ratings.
In another example, an e-commerce company utilized text analytics to analyze customer interactions across various channels. By identifying common customer concerns and predictive question examples, the company streamlined its knowledge base and support materials to address recurring issues proactively. This reduced support tickets and allowed for quicker issue resolution.
A final example might involve a financial services provider using predictive analytics to enhance its customer segmentation and target marketing efforts. The company identified distinct customer segments and tailored its communication strategies accordingly. This personalized approach resulted in increased customer engagement and higher retention rates.
Importance of Predictive Analytics
Predictive analytics plays an undoubtedly vital role in call center operations, as it enables businesses to optimize resources and improve customer experiences. Centers that capture the power of advanced algorithms and data analysis techniques are able to anticipate future trends, identify areas for improvement, and implement targeted solutions to enhance overall performance.
One of the primary benefits of predictive analytics in call centers is its ability to improve workforce management predictions. This works by analyzing historical data on call volumes and agent availability to forecast future workloads and staffing requirements. It allows call centers to optimize agent scheduling so that they can schedule the right number of people on the right days and at the right times, which can prevent over- or under-staffing.
Call center data analysis prediction also plays a crucial role in enhancing customer experiences. By analyzing customer interactions and feedback, call centers can identify patterns and trends that indicate potential areas for improvement. This may involve refining communication strategies or implementing new tools to streamline processes.
Predictive analytics can also help in maximizing call center efficiency by identifying the factors that contribute to reduced call handling times and increased customer satisfaction. Taking these factors into account, call centers can develop targeted training programs for agents, implement process improvements, and make informed decisions on technology investments.
The importance of predictive analytics for call centers, therefore, lies in its ability to provide actionable insights that drive continuous improvement and optimization. It enables centers to optimize workforce management, improve customer experiences, and much more. When utilized correctly, predictive analytics can drastically improve an organization’s bottom line.
Authenticx was specifically designed for healthcare companies looking to leverage analytics to improve the customer journey. The solution listens to, analyzes, and activates customer voices to identify issues in their experience and equip users with the tools they need to improve operations.